‘Blog’
Technical Writing and its ‘Hierarchy of Needs’
Wednesday, February 2nd, 2022
Technical writing is hard to do well and it’s also a bit different from other types of writing. While good technical writing has no strict definition I do think there is a kind of ‘hierarchy of needs’ that defines it. I’m not sure this is complete or perfect but I find categorizing to be useful.
L1 – Writing Clearly
The author writes in a way that accurately represents the information they want to convey. Sentences have a clear purpose. The structure of the text flows from point to point.
L2 – Explaining Well (Logos in rhetorical theory)
The author breaks their argument down into logical blocks that build on one another to make complex ideas easier to understand. When done well, this almost always involves (a) short, inline examples to ground abstract ideas and (b) a concise and logical flow through the argument which does not repeat other than for grammatical effect or flip-flop between points.
L3 – Style
The author uses different turns of phrase, switches in person, different grammatical structures, humor, etc. to make their writing more interesting to read. Good style keeps the reader engaged. You know it when you see it as the ideas flow more easily into your mind. Really good style even evokes an emotion of its own. By contrast, an author can write clearly and explain well, but in a way that feels monotonous or even boring.
L4 – Evoking Emotion (Pathos in rhetorical theory)
I think this is the most advanced and also the most powerful particularly where it inspires the reader to take action based on your words through an emotional argument. To take an example, Martin Kleppmann’s turning the database inside out inspired a whole generation of software engineers to rethink how they build systems. Tim or Kris’ humor works in a different but equally effective way. Other appeals include establishing a connection with the reader, grounding in a subculture that the author and reader belong to, establishing credibility (ethos), highlighting where they are missing out on (FOMO), influencing through knowing and opinionated command of the content. There are many more.
The use of pathos (sadly) doesn’t always imply logos, often there are logical fallacies used even in technical writing. Writing is so much more powerful if both are used together.
Related: livingston county, ny car accident, russell poole a cop we should insist on, jobs in salem oregon craigslist, petrina johnson and robert crisp still together, is aunt capitalized in spanish, log cabin kits reno nv, virginia covid sick leave 2021, ette place, can i fly with a cough coronavirus, mobile homes for rent in claremore, ok, avianca covid test requirements el salvador, michelle branca lee, population of aberystwyth, evergreen job requisition successfactors, terraria seed for jungle temple,Related: lamar hunt family tree, who is professor waldman in frankenstein, modern farmhouse virtual tour matterport, christus health employee benefits, daniel m melber seattle, dover banger racing fixtures 2022, is ukee washington in quarantine, roadtrek zion used for sale, how to clean old military uniforms, 2016 forest river sandpiper destination, lymphatic massage swindon, rehome french bulldog, how many copies has minecraft sold 2022, communion wine sainsbury’s, jen herro age,Related: bar louie voodoo sauce recipe, your tickets finished uploading and are now processing seatgeek, lord beaverbrook net worth, best photography locations near worcester, ma, lelit elizabeth v3 vs profitec pro 300, binghamton housing authority executive director, jasper county inmate roster, how to bleed air from ice maker line, gary edwards obituary, luke bryan farm tour 2022, upnorthlive car accident today, referee march madness, wslregisterdistribution failed with error 0xc0000005, dorset police helicopter tracker, bustle horoscope 2022,Related: cbp training academy housing, grants for barn restoration, tendaji lathan mother, fell compassionate analogy, how old was sandra bullock in hope floats, ejmr finance rumor, medders funeral home crossett, arkansas obituaries, tricare east corrected claims, how to change reporting lines in powerpoint org chart, cheap apartments winston salem, miami beach 1990s nightclubs, healy foundation new mexico, alone show worst injuries, cypress, tx weather monthly, dallas piano competition,Related: cyclace exercise bike manual, what is smart card pairing on my mac, octopus benefits testosterone, average mile time for college soccer players, clearwater, florida obituaries 2022, green goblin laugh sound effect, wrat 4 scoring guide, bobby flay restaurants los angeles, christian spiritual retreats near me, are pending charges included in total balance amex, sonic boom today california, keplinger funeral home obituaries, propane house explosion, owl attack human injuries, where is george from what the hales,Related: how to turn off water blur in the forest, homes for rent by owner vineland, nj, findlay city schools staff directory, school district of philadelphia climate manager salary, 3 week itinerary italy and switzerland, the brand closet employee login kate spade, madison county, va obituaries, relocation assistance jp morgan, columbus zoo arctic fox anana death, charles joshua powell, paloma tree fataar, mass state retirement chart group 2, flamingos restaurant menu, is timothy busfield in a wheelchair, houses for rent in kissimmee under $1200,Related: what happened to larry hinson, kleenheat contact number, elemental grind game code, tauranga hospital orthopaedics, shippensburg university basketball, former komo news reporters, command style coaching pros and cons, barrett jackson auction, haycombe crematorium schedule, tuscany wedding packages, 765 rockbridge rd, montecito, ca 93108, guns n’ roses tribute band massachusetts, birchen clough scramble, beaver dam raceway tickets, zivilisatorisches hexagon beispiel irak,Related: barry soper ex wife, con que puedo sustituir el nopal en una dieta, goffs school teachers, noodler’s ink controversy, marriott staff directory, civil standby colorado, waterfront homes for sale yamba, nsw, quartz crystal cave oregon, thank you to church family after funeral, black funeral homes in fort pierce, fl, 54 bus on sunday, iterate through list of dictionaries python, how does hsa work with child support, self mutilation waiver air force, splinter removal kit walgreens,Related: usps background check red flag, where to find geodes in south carolina, argyle baseball roster 2021, alternative to gruyere cheese in dauphinoise, how to make multichrome eyeshadow, the lighthouse mermaid scene, bob uecker siblings, is army of two 40th day backwards compatible on xbox one, skinwalker deer video, gila county jail payson, az mugshots, , firefighter powerpoint presentations, casey johnson obituary, how to fix peeling shirt printing, cabrini cardiologists,Related: how to allocate more ram to sims 4, optimo cigars expiration date, how did antoinette chanel die, calories in 1 tbsp brown sugar, joey rourke cause of death, minecraft but you can combine any items mod, meijer cake catalog, amado carrillo fuentes’ death, spencer reid maeve, is mrcp recognised in canada, mitsuhiko kanekatsu’s bww vin decoder, le desenvoutement dure combien de temps, platform technologies syllabus ched, aaron jones nicknames, iron ii chromate formula,Related: passaic high school staff directory, arizona modern furniture, cameron rachel hamill, ahimelech and abimelech, underrail quest order, worcester arrests yesterday, ovary pain when walking, boronia beach penguins, small wedding venues virginia, fm 590pp non dot urine labcorp, meghan markle mean to charlotte, etsy removable wall murals, john bolling descendants, central bedfordshire tidy tip booking, spotify premium family invite,Related: big john studd vietnam, what replaced redken diamond oil, cooper creek campground georgia, taupo death notices 2021, mecklenburg county candidates 2022, mayfield middle school bell schedule, anthurium jenmanii variegated, where does lolo jones live now, farruko health problems, alamo heights football roster, shortened descriptor example, what gyms accept issa certification, st cuthbert’s school, newcastle staff list, alameda county police scanner, who died on shameless in real life,Related: john lippoth obituary, shopping in bay st louis, jet2 advert 2020 actress, does evening primrose oil make your breasts bigger, aws glue api example, wheatgrass histamine intolerance, jetstar vaccination policy within australia, all of the following are presidential roles except, reasons for failure of moon treaty, which city in new zealand has the highest crime rate, medical surgical assessment exam quizlet, ponchatoula, louisiana murders, brands like blackbough swim, , coast g25 flashlight manual,Related: how to take apart a kohler kitchen faucet, was steven seagal a navy seal, native plants for erosion control, savage 93 22 mag magazine problems, jerome ghost tour groupon, ford edge throttle body recall, cellular network not available for voice calls moto g7, operation odessa where are they now, sea of thieves can’t change resolution, 1950 craftsman tool catalog, 2010 kubota rtv 900 specs, roseburg oregon tv channels, mastidane puppies for sale near me, tony robinson nfl philadelphia, sisters of st mary peekskill, ny,Related: new construction homes under $250k near me, texas southern university sororities and fraternities, tavistock police news, holly pollard net worth, ohio liquor license search, what is an example of mutualism in the tropical rainforest, watkins mill youth detention center, funny baseball awards, grace and frankie when did mallory have twins, martha paiz fogerty, what does cps look for in a home study, gibson l3 for sale, willow pump blinking red while charging, roane county recent obituaries, difference between scabies and ringworm,Related: sample motion for temporary orders massachusetts, eybl team tryouts 2022, is left axis deviation ecg dangerous, 30 day weather forecast for montana, cuanto tarda en secar la tinta para madera, remove speed limiter on mobility scooter, grant parish school board pay scale, quien es el esposo de coco march, security jobs paying $30 an hour, dreamline shower base drain size, pgh laparoscopic surgery cost, mitchell goldhar wife, union county section 8 plainfield, nj, jackson high school basketball schedule 2021, south africa surnames,Related: wasserstein private equity, walgreens nationals logo lawsuit, triton boats apparel, how to sneak your phone in a jail visit, albertsons software engineer salary near berlin, aziende biomediche svizzera, how did richard karn lose weight, danny kelly wife, who is the mom in the liberty mutual nostalgia commercial, where does martina navratilova live in miami, normal cranial vault asymmetry index, nextamz wireless thermometer manual, 2019 california green building standards code dwg, can ‘t smile after septoplasty, katie lange age,Related: men’s minimal coverage swimwear, how to defer a ticket in king county, is the bubble room haunted, levi strauss foundation executive director salary, joe zolper t shirts, bladen county crime, laporte county assessor property search, elliot hospital cafeteria menu, whitby to scarborough boat trip, usc applied data science faculty, what does it mean when a man calls you boss, 3 types of licence issued under the licensing act 2003, who is letitia james parents, joanna and shariece clark update 2021, wa lottery app says please see lottery,Related: conservative razor companies, the parsons family murders, fishtail palm poisonous to dogs, do you need a license to breed snakes in california, 12 gauge round ball, whodini vh1 hip hop honors performance, ke huy quan wife, brandon davis obituary, mike and kelly bowling divorce, police chase in plano tx today, kyker funeral home harriman, tn obituaries, luscombe 8a bush plane, return enchantment from graveyard standard, bend, oregon mugshots 2021, saintsations auditions 2021,Related: pets at home photo competition 2021, southern district of texas, list of funerals at three counties crematorium, zero emission vehicle companies, eml newcastle address, gila county mugshots, steve reevis death cause, jupiter pizza menu nutrition, anchorage airport live camera, ffxiv too close to home report to central shroud, ansa keyboard shortcuts, watertown building department, nys dmv registration renewal form, zaandam refurbishment 2020, how old is kelly austin,Related: 2022 nfl mock draft simulator, how long does air duster stay in your system, osha does not approve individual states true or false, nebraska missing persons, lake of the woods real estate oregon, western intercollegiate golf leaderboard, jefferson hospital patient information, housing authorities absorbing vouchers, terri halperin married, slimline tumble dryer 45cm wide currys, what is the name of c3n4 compound name, what is the first step of the spider method, which three statements are accurate about debug logs, where did muhammad ali live before he died, dysautonomia covid vaccine reaction,Related: top 10 dangerous caste in pakistan, panda express calorie calculator, everlast gym opening times, shooting in rialto today, can i take shilajit with coffee, short badass military quotes, 3 grand trines in natal chart, pennsbury high school football, accidentally cooked plastic with food, the ancient and noble house of black revolution fanfiction, taurus man fantasy gemini woman, sturm funeral home, sleepy eye obituaries, trevor siemian career earnings, east alton police blotter, ashley county warrant list,Related: khnadya skye nelson age, mary elizabeth mcdonough, cheryl araujo daughters where are they now, ako prejavuje lasku vodnar, university of miami volleyball coach email, why do monkeys reject their babies, superintendent humble isd, when do orioles leave michigan, scottish terrier bite force psi, unsolved murders in fayetteville, nc, dirk diggler prosthetic picture, skytech m1000 mouse software, bishopric youth discussion handbook, current road conditions boulia to birdsville, filing a false order of protection illinois,Related: general jack keane girlfriend, who killed tyler in a dark place spoiler, how much bigger is earth than mars in km, texas franchise tax public information report 2022, asgore fight simulator github, gm financial late payment removal, contact alo yoga customer service, west ashley accident today, tufts baseball recruiting questionnaire, tatum coffey wedding, nen ability generator, gannon shepherd wife, sydney swans academy players 2021, who was ogden stiers partner, south dakota gun laws for non residents,Related: new york obituaries archives, bungalows for sale in waltham abbey, commodification of hawaiian culture, dallas construction projects 2022, is nico de boinville married, who is betty klimenko husband, thigh tattoo hurts to walk, otto ohlendorf descendants, menards coming to gaylord, mi, jamie oliver 123 traybake, how did alejandro family die in sicario, purdue university dorms, starting specific gravity for moonshine mash, explain col using at least three descriptors, grants for private practice counseling,Related: am i attractive to guys quiz buzzfeed, how long to walk around port lympne zoo, lancaster county school district salary schedule, adjectives to describe ray bradbury’s life, tesla model 3 grinding noise, muscle twitching all over body at rest forum, are rachel and mitchell moranis twins, johnny depp horoscope, screen mirroring windows 11 to tv, is harold perrineau really in a wheelchair, top hernia surgeons long island, mdot executive director, barrow neurosurgery fellows, world’s strictest parents tamsin update, nigel clough wife,Related: tiny times 4 ending explained, nothing to declare australia nadia, expert mode terraria boss drops, fran lebowitz ellen lebowitz, muddy paws rescue omaha, gillespie county election results 2022, ralph capone jr, harry and meghan snubbed by spotify, john hammergren family, celebration of life venues portland oregon, american manchester terrier club rescue, did kelly preston have chemotherapy for her cancer, why did they stop selling jolly ranchers in the uk, juegos para mayores de 18, don gordon wife,Related: iowa 1st congressional district 2022, st francis hospital cafeteria menu, chrysler dealer code lookup, what happens when you win a microsoft sweepstakes, warzone unlock all tool discord, peterson and williams funeral home obituaries, brogo dam canoe hire, halifax courier archive obituaries, does catherine oxenberg have a royal title, navarre press arrests, isabelle townsend today, warble home remedy, what to wear to a wardruna concert, what to say when someone says trust me, xkw1 switch hack 2021,Related: things to do between grand canyon and moab, royal burger bernie mac, kennesaw mountain high school news, palm harbor homes class action lawsuit, senior open qualifying 2022 entry form, pickleball courts bellevue, franklin county busted, fedex ground rates per pound, yousif tlaib, how tall was clint walker’s twin sister lucy, curtis pilot polo net worth, ohio college of podiatric medicine ranking, east hampton, ct woman found dead, christus health employee benefits, how much weight can a 2×3 support horizontally,Related: shelby 5101 trailer vin location, illegal eagles christian, lynne thigpen obituary, what does tom hagen say to vincenzo pentangeli, houses for rent statham, ga, quien fue azeneth, tree preservation order map cardiff, crooked lake bc cabin for sale, nexrad level 3 data feed, rick stacy morning show today, mary mcgowan attorney virginia, roan mountain state park trail map, jasper county ga obituaries, zakros ancient tablet, how to use debit card before it arrives,Related: megan rapinoe fan mail address, ashtabula county sheriff reports, shooting in cookeville, tn today, disadvantages of chemical synapses, haysville, ks warrant search, eccles tram stop to aj bell stadium, moderation management pdf, what happens if you eat expired pez candy, coliban potato substitute, was johnny cash museum damaged in explosion, which camp buddy character are you quiz, north coast wine transport, quadratic graph calculator with points, lollapalooza 2022 lineup, acacia acuminata queensland,Related: omma testing requirements, benchmade infidel upgrades, tesco policies and procedures, penn state 1987 football roster, error during websocket handshake: unexpected response code: 404, pebble shore lake montana map, plastic surgeon st vincent’s private hospital, st james high school football record, lind family funeral home obituaries, wbbj crime stoppers, glamrock freddy voice, lipscomb university lectureship, watertown building department, how much health does templar have destiny 2, choking on liquids after thyroidectomy,Related: oldies concerts 2022 california, does one love mean i love you, pickering creek reservoir boating, absolute roughness of stainless steel, joanne froggatt downton abbey, ace model 1000 garbage disposal installation manual, city of tempe setback requirements, how many times can 8 go into 2, kirk cousins house, past mayors of danbury, ct, apartment comparison spreadsheet google sheets, what size easel do i need for a 16×20 canvas, dell s2721qs calibration settings, new york to miami sleeper train, does heinz simply ketchup taste different,Related: boshamer stadium rules, lenny breau daughter, quarter horses for sale in massachusetts, south ribble council contact number, aics thumbhole upgrade, can an ovarian cyst cause leukocytes in urine, miami airport to eden roc hotel, travelodge saver rate cancellation, new balance commercial baseball player, in texas party politics today quizlet, north carolina symphony musicians, how far can a bobcat jump horizontally, nfl communications staff directory, carvelli restaurant group, accident on 223 adrian, mi today,Designing Event Driven Systems – Summary of Arguments
Thursday, October 4th, 2018
This post provides a terse summary of the high-level arguments addressed in my book.
Why Change is Needed
Technology has changed:
- Partitioned/Replayable logs provide previously unattainable levels of throughput (up to Terabit/s), storage (up to PB) and high availability.
- Stateful Stream Processors include a rich suite of utilities for handling Streams, Tables, Joins, Buffering of late events (important in asynchronous communication), state management. These tools interface directly with business logic. Transactions tie streams and state together efficiently.
- Kafka Streams and KSQL are DSLs which can be run as standalone clusters, or embedded into applications and services directly. The latter approach makes streaming an API, interfacing inbound and outbound streams directly into your code.
Businesses need asynchronicity:
- Businesses are a collection of people, teams and departments performing a wide range of functions, backed by technology. Teams need to work asynchronously with respect to one another to be efficient.
- Many business processes are inherently asynchronous, for example shipping a parcel from a warehouse to a user’s door.
- A business may start as a website, where the front end makes synchronous calls to backend services, but as it grows the web of synchronous calls tightly couple services together at runtime. Event-based methods reverse this, decoupling systems in time and allowing them to evolve independently of one another.
A message broker has notable benefits:
- It flips control of routing, so a sender does not know who receives a message, and there may be many different receivers (pub/sub). This makes the system pluggable, as the producer is decoupled from the potentially many consumers.
- Load and scalability become a concern of the broker, not the source system.
- There is no requirement for backpressure. The receiver defines their own flow control.
Systems still require Request Response
- Whilst many systems are built entirely-event driven, request-response protocols remain the best choice for many use cases. The rule of thumb is: use request-response for intra-system communication particularly queries or lookups (customers, shopping carts, DNS), use events for state changes and inter-system communication (changes to business facts that are needed beyond the scope of the originating system).
Data-on-the-outside is different:
- In service-based ecosystems the data that services share is very different to the data they keep inside their service boundary. Outside data is harder to change, but it has more value in a holistic sense.
- The events services share form a journal, or ‘Shared Narrative’, describing exactly how your business evolved over time.
Databases aren’t well shared:
- Databases have rich interfaces that couple them tightly with the programs that use them. This makes them useful tools for data manipulation and storage, but poor tools for data integration.
- Shared databases form a bottleneck (performance, operability, storage etc.).
Data Services are still “databases”:
- A database wrapped in a service interface still suffers from many of the issues seen with shared databases (The Integration Database Antipattern). Either it provides all the functionality you need (becoming a homegrown database) or it provides a mechanism for extracting that data and moving it (becoming a homegrown replayable log).
Data movement is inevitable as ecosystems grow.
- The core datasets of any large business end up being distributed to the majority of applications.
- Messaging moves data from a tightly coupled place (the originating service) to a loosely coupled place (the service that is using the data). Because this gives teams more freedom (operationally, data enrichment, processing), it tends to be where they eventually end up.
Why Event Streaming
Events should be 1st Class Entities:
- Events are two things: (a) a notification and (b) a state transfer. The former leads to stateless architectures, the latter to stateful architectures. Both are useful.
- Events become a Shared Narrative describing the evolution of the business over time: When used with a replayable log, service interactions create a journal that describes everything a business does, one event at a time. This journal is useful for audit, replay (event sourcing) and debugging inter-service issues.
- Event-Driven Architectures move data to wherever it is needed: Traditional services are about isolating functionality that can be called upon and reused. Event-Driven architectures are about moving data to code, be it a different process, geography, disconnected device etc. Companies need both. The larger and more complex a system gets, the more it needs to replicate state.
Messaging is the most decoupled form of communication:
- Coupling relates to a combination of (a) data, (b) function and (c) operability
- Businesses have core datasets: these provide a base level of unavoidable coupling.
- Messaging moves this data from a highly coupled source to a loosely coupled destination which gives destination services control.
A Replayable Log turns ‘Ephemeral Messaging’ into ‘Messaging that Remembers’:
- Replayable logs can hold large, “Canonical” datasets where anyone can access them.
- You don’t ‘query’ a log in the traditional sense. You extract the data and create a view, in a cache or database of your own, or you process it in flight. The replayable log provides a central reference. This pattern gives each service the “slack” they need to iterate and change, as well as fitting the ‘derived view’ to the problem they need to solve.
Replayable Logs work better at keeping datasets in sync across a company:
- Data that is copied around a company can be hard to keep in sync. The different copies have a tendency to slowly diverge over time. Use of messaging in industry has highlighted this.
- If messaging ‘remembers’, it’s easier to stay in sync. The back-catalogue of data—the source of truth–is readily available.
- Streaming encourages derived views to be frequently re-derived. This keeps them close to the data in the log.
Replayable logs lead to Polyglot Views:
- There is no one-size-fits-all in data technology.
- Logs let you have many different data technologies, or data representations, sourced from the same place.
In Event-Driven Systems the Data Layer isn’t static
- In traditional applications the data layer is a database that is queried. In event-driven systems the data layer is a stream processor that prepares and coalesces data into a single event stream for ingest by a service or function.
- KSQL can be used as a data preparation layer that sits apart from the business functionality. KStreams can be used to embed the same functionality into a service.
- The streaming approach removes shared state (for example a database shared by different processes) allowing systems to scale without contention.
The ‘Database Inside Out’ analogy is useful when applied at cross-team or company scales:
- A streaming system can be thought of as a database turned inside out. A commit log and a a set of materialized views, caches and indexes created in different datastores or in the streaming system itself. This leads to two benefits.
- Data locality is used to increase performance: data is streamed to where it is needed, in a different application, a different geography, a different platform, etc.
- Data locality is used to increase autonomy: Each view can be controlled independently of the central log.
- At company scales this pattern works well because it carefully balances the need to centralize data (to keep it accurate), with the need to decentralise data access (to keep the organisation moving).
Streaming is a State of Mind:
- Databases, Request-response protocols and imperative programming lead us to think in blocking calls and command and control structures. Thinking of a business solely in this way is flawed.
- The streaming mindset starts by asking “what happens in the real world?” and “how does the real world evolve in time?” The business process is then modelled as a set of continuously computing functions driven by these real-world events.
- Request-response is about displaying information to users. Batch processing is about offline reporting. Streaming is about everything that happens in between.
The Streaming Way:
- Broadcast events
- Cache shared datasets in the log and make them discoverable.
- Let users manipulate event streams directly (e.g., with a streaming engine like KSQL)
- Drive simple microservices or FaaS, or create use-case-specific views in a database of your choice
The various points above lead to a set of broader principles that summarise the properties we expect in this type of system:
The WIRED Principles
Windowed: Reason accurately about an asynchronous world.
Immutable: Build on a replayable narrative of events.
Reactive: Be asynchronous, elastic & responsive.
Evolutionary: Decouple. Be pluggable. Use canonical event streams.
Data-Enabled: Move data to services and keep it in sync.
REST Request-Response Gateway
Thursday, June 7th, 2018
This post outlines how you might create a Request-Response Gateway in Kafka using the good old correlation ID trick and a shared response topic. It’s just a sketch. I haven’t tried it out.
A Rest Gateway provides an efficient Request-Response bridge to Kafka. This is in some ways a logical extension of the REST Proxy, wrapping the concepts of both a request and a response.
What problem does it solve?
- Allows you to contact a service, and get a response back, for example:
- to display the contents of the user’s shopping basket
- to validate and create a new order.
- Access many different services, with their implementation abstracted behind a topic name.
- Simple Restful interface removes the need for asynchronous programming front-side of the gateway.
So you may wonder: Why not simply expose a REST interface on a Service directly? The gateway lets you access many different services, and the topic abstraction provides a level of indirection in much the same way that service discovery does in a traditional request-response architecture. So backend services can be scaled out, instances taken down for maintenance etc, all behind the topic abstraction. In addition the Gateway can provide observability metrics etc in much the same way as a service mesh does.
You may also wonder: Do I really want to do request response in Kafka? For commands, which are typically business events that have a return value, there is a good argument for doing this in Kafka. The command is a business event and is typically something you want a record of. For queries it is different as there is little benefit to using a broker, there is no need for broadcast and there is no need for retention, so this offers little value over a point-to-point interface like a HTTP request. So the latter case we wouldn’t recommend this approach over say HTTP, but it is still useful for advocates who want a single transport and value that over the redundancy of using a broker for request response (and yes these people exist).
This pattern can be extended to be a sidecar rather than a gateway also (although the number of response topics could potentially become an issue in an architecture with many sidecars).
Implementation
Above we have a gateway running three instances, there are three services: Orders, Customer and Basket. Each service has a dedicated request topic that maps to that entity. There is a single response topic dedicated to the Gateway.
The gateway is configured to support different services, each taking 1 request topic and 1 response topic.
Imagine we POST and Order and expect confirmation back from the Orders service that it was saved. This work as follows:
- The HTTP request arrives at one node in the Gateway. It is assigned a correlation ID.
- The correlation ID is derived so that it hashes to a partition of the response topic owned by this gateway node (we need this to route the request back to the correct instance). Alternatively a random correlation id could be assigned and the request forwarded to the gateway node that owns the corresponding partition of the response topic.
- The request is tagged with a unique correlation ID and the name of the gateway response topic (each gateway has a dedicated response topic) then forwarded to the Orders Topic. The HTTP request is then parked in the webserver.
- The Orders Service processes the request and replies on the supplied response topic (i.e. the response topic of the REST Gateway), including the correlation ID as the key of the response message. When the REST Gateway receives the response, it extracts the correlation ID key and uses it to unblock the outstanding request so it responds to the user HTTP request.
Exactly the same process can be used for GET requests, although providing streaming GETs will require some form of batch markers or similar, which would be awkward for services to implement probably necessitating a client-side API.
If partitions move, whist requests are outstanding, they will timeout. We could work around this but it is likely acceptable for an initial version.
This is very similar to the way the OrdersService works in the Microservice Examples
Event-Driven Variant
When using an event driven architecture via event collaboration, responses aren’t based on a correlation id they are based on the event state, so for example we might submit orders, then respond once they are in a state of VALIDATED. The most common way to implement this is with CQRS.
Websocket Variant
Some users might prefer a websocket so that the response can trigger action rather than polling the gateway. Implementing a websocket interface is slightly more complex as you can’t use the queryable state API to redirect requests in the same way that you can with REST. There needs to be some table that maps (RequestId->Websocket(Client-Server)) which is used to ‘discover’ which node in the gateway has the websocket connection for some particular response.
Slides from Craft Meetup
Wednesday, May 9th, 2018
The slides for the Craft Meetup can be found here.
Book: Designing Event Driven Systems
Friday, April 27th, 2018
I wrote a book: Designing Event Driven Systems
MOBI (Kindle)
Building Event Driven Services with Kafka Streams (Kafka Summit Edition)
Monday, April 23rd, 2018
The Kafka Summit version of this talk is more practical and includes code examples which walk though how to build a streaming application with Kafka Streams.
Slides fo NDC – The Data Dichotomy
Friday, January 19th, 2018
Ben Stopford explains why understanding and accepting this dichotomy is an important part of designing service-based systems at any significant scale. Ben looks at how companies make use of a shared, immutable sequence of records to balance data that sits inside their services with data that is shared, an approach that allows the likes of Uber, Netflix, and LinkedIn to scale to millions of events per second.
Ben concludes by examining the potential of stream processors as a mechanism for joining significant, event-driven datasets across a whole host of services and explains why stream processing provides much of the benefits of data warehousing but without the same degree of centralization.
Handling GDPR: How to make Kafka Forget
Monday, December 4th, 2017
If you follow the press around Kafka you’ll probably know it’s pretty good at tracking and retaining messages, but sometimes removing messages is important too. GDPR is a good example of this as, amongst other things, it includes the right to be forgotten. This begs a very obvious question: how do you delete arbitrary data from Kafka? It’s an immutable log after all.
As it happens Kafka is a pretty good fit for GDPR as, along with the right to be forgotten, users also have the right to request a copy of their personal data. Companies are also required to keep detailed records of what data is used for — a requirement where recording and tracking the messages that move from application to application is a boon.
How do you delete (or redact) data from Kafka?
The simplest way to remove messages from Kafka is to simply let them expire. By default Kafka will keep data for two weeks and you can tune this as required. There is also an Admin API that lets you delete messages explicitly if they are older than some specified time or offset. But what if we are keeping data in the log for a longer period of time, say for Event Sourcing use cases or as a source of truth? For this you can make use of Compacted Topics, which allow messages to be explicitly deleted or replaced by key.
Data isn’t removed from Compacted Topics in the same way as say a relational database. Instead Kafka uses a mechanism closer to those used by Cassandra and HBase where records are marked for removal then later deleted when the compaction process runs.
To make use of this you configure the topic to be compacted and then send a delete event (by sending a null message, with the key of the message you want to delete). When compaction runs the message will be deleted forever.
//Create a record in a compacted topic in kafka producer.send(new ProducerRecord(CUSTOMERS_TOPIC, “Donald Trump”, “Job: Head of the Free World, Address: The White House”)); //Mark that record for deletion when compaction runs producer.send(new ProducerRecord(CUSTOMERS_TOPIC, “Donald Trump”, null));
If the key of the topic is something other than the CustomerId then you need some process to map the two. So for example if you have a topic of Orders, then you need a mapping of Customer->OrderId held somewhere. Then to ‘forget’ a customer simply lookup their Orders and either explicitly delete them, or alternatively redact any customer information they contain. You can do this in a KStreams job with a State Store or alternatively roll your own.
There is a more unusual case where the key (which Kafka uses for ordering) is completely different to the key you want to be able to delete by. Let’s say that, for some reason, you need to key your Orders by ProductId. This wouldn’t be fine-grained enough to let you delete Orders for individual customers so the simple method above wouldn’t work. You can still achieve this by using a key that is a composite of the two: [ProductId][CustomerId] then using a custom partitioner in the Producer (see the Producer Config: “partitioner.class”) which extracts the ProductId and uses only that subsection for partitioning. Then you can delete messages using the mechanism discussed earlier using the [ProductId][CustomerId] pair as the key.
What about the databases that I read data from or push data to?
Quite often you’ll be in a pipeline where Kafka is moving data from one database to another using Kafka Connectors. In this case you need to delete the record in the originating database and have that propagate through Kafka to any Connect Sinks you have downstream. If you’re using CDC this will just work: the delete will be picked up by the source Connector, propagated through Kafka and deleted in the sinks. If you’re not using a CDC enabled connector you’ll need some custom mechanism for managing deletes.
How long does Compaction take to delete a message?
By default compaction will run periodically and won’t give you a clear indication of when a message will be deleted. Fortunately you can tweak the settings for stricter guarantees. The best way to do this is to configure the compaction process to run continuously, then add a rate limit so that it doesn’t doesn’t affect the rest of the system unduly:
# Ensure compaction runs continuously with a very low cleanable ratio log.cleaner.min.cleanable.ratio = 0.00001 # Set a limit on compaction so there is bandwidth for regular activities log.cleaner.io.max.bytes.per.second=1000000
Setting the cleanable ratio to 0 would make compaction run continuously. A small, positive value is used here, so the cleaner doesn’t execute if there is nothing to clean, but will kick in quickly as soon as there is. A sensible value for the log cleaner max I/O is [max I/O of disk subsystem] x 0.1 / [number of compacted partitions]. So say this computes to 1MB/s then a topic of 100GB will clean removed entries within 28 hours. Obviously you can tune this value to get the desired guarantees.
One final consideration is that partitions in Kafka are made from a set of files, called segments, and the latest segment (the one being written to) isn’t considered for compaction. This means that a low throughput topic might accumulate messages in the latest segment for quite some time before rolling, and compaction kicking in. To address this we can force the segment to roll after a defined period of time. For example log.roll.hours=24 would force segments to roll every day if it hasn’t already met its size limit.
Tuning and Monitoring
There are a number of configurations for tuning the compactor (see properties log.cleaner.* in the docs) and the compaction process publishes JMX metrics regarding its progress. Finally you can actually set a topic to be both compacted and have an expiry (an undocumented feature) so data is never held longer than the expiry time.
In Summary
Kafka provides immutable topics where entries are expired after some configured time, compacted topics where messages with specific keys can be flagged for deletion and the ability to propagate deletes from database to database with CDC enabled Connectors.
What could academia or industry could do (short or long term) to promote more collaboration?
Saturday, October 14th, 2017
I did a little poll of friends and colleagues about this question. Here are some of the answers which I found quite thought provoking:
I’m a recovering academic from many years ago. I feel like I have some perspective on graduate/research departments in computer science, even though I am sure things have changed a little since I was in grad school.
One problem I saw is that a ton of the research done in Universities in computer science (outside areas like quantum computing, etc) lags behind industry. A lot of graduate students in Software Engineering worked on projects that capable companies had already solved or that a senior industry developer could solve in a few weeks.
I also see a lot of graduate student project where they end up “building a tool” except the tool ends up being something nobody would ever use.
Every single one of those kinds of projects destroys the credibility of academics with industry.
A victory for academics seems to be publication or assembling statistical evidence for an assertion. I get it but nobody in industry cares about those things. Nobody. Change your goalposts and align them with industry if you want to collaborate with industry.
I also think there is huge overlap between graduate student research and startups. Lets say I’m 24 years old, and I think I have an idea to change the world with technology. Instead of doing it at the University for a M.Sc I can just get some investment and build a startup (even without a business plan sometimes).
If academics want collaboration they need to be brutally honest with themselves and get more focused while facing where they sit today. The software being written inside Universities often sucks. The research often moves too slowly. Startups are the innovators. The kinds of evidence and assertions being “proven” in academia are mostly uninteresting. The outputs like publications are only read by other academics.
It might hurt but if you want credibility, cancel some of that crap. Work in the future, not in the past, understand your strengths and weaknesses and play to your strengths, change your goals to deliver outputs that are really consumable…
Its a lot to ask, so I don’t see any of that happening…
My company, engages quite a lot with academia, and even runs an Institute partly for this. The following is a bit of a brain-dump.
Within the institute we employ an academic-in-residence (Carlota Perez.) This is to explicitly support and sponsor work that we think is valuable and should be completed. In this case, to help her finish her second book. The institute also runs a fellowship programme. This is broadly defined to attract individuals with ideas and talent to offer them a network and opportunities, supported by a stipend. We explicitly define this quite broadly to allow people who may not want to start businesses to find value.
Obviously we’re interested in finding people who want to start businesses, but we keep that distinct from the fellowship to allow more far-reaching visions space to grow, at least a little. If fellows do want to found a business, and are capable of it, then we draw them into and support them in that.
We’re looking to participate more in academic-industry think-tanks, and other bodies. We individually connect to people in these bodies, and in academia, a lot in workshops we run. Mostly to generate ideas and explore spaces.
Finally, we read a lot of papers.
In our view, this is a start, but not enough. We are doing a little to sponsor the development of ideas within academia, via Carlota Perez, and we’re allowing people to start research projects in the fellowship. But we want to help with more execution and scale. We’ve tried to partner with some universities, but we find that they’re not commercially-focused enough to support us in raising the capital to actually execute with. They want to provide ideas, we provide execution, and capital appears by magic. We need a bit more than that.
I was affiliated with [Top UK University] for a time and here is my top-2 list of difficulties:
– IP: the university makes it really hard to separate the IP between work done during the collaboration vs work done in the day job (industry). The amount of paperwork is typical of a bureaucratic institution. Turn off for many people (why bother).
– IP again: this is slightly tangential to the original question and is more related to a different kind of industry-academia collaboration, one where the prof does a startup while in academia. [Top UK University] for example had a policy that 50% of the equity of the startup belonged to [Top UK University]. That number is huge. Prevents other VCs from investing in the startup. Guarantees that basically no one will do a serious startup. A more comparable number in leading US universities like Stanford is 2-5%. There were creative ways around that, but it was a grey area legally. Again, why would one bother going through the hoops. It’s easier to just not deal with academia at all.
My suggestion would be that industry and academia need to develop more understanding of, and respect for, each other’s needs and incentives. To put it bluntly, the career demands are very different: industry people need to ship products that customers care about, while academics need to publish papers in good venues. With those different incentives come different timelines for working (industry thinks about shipping quickly and long-term maintenance; academia thinks about big ideas for the future, but doesn’t care about the code once the paper is published), different prioritisation of aspects of the work (e.g. testing), etc. Of course those are over-simplified caricatures, but I hope you get the idea.
I don’t think one is better than the other — they are just different, and for a collaboration to be productive, I think there needs to be mutual understanding and empathy for these different needs. People who have only worked in one of the two may get frustrated with people from the other camp, feeling that they just “don’t get what’s important” (because indeed different things are important).
Caveat: I’m still affiliated with various academic advisory boards so am somewhat biased by the progress we’re making. A few personal comments / observations:
– Although academia has shifted slightly to focus more on “impact” not just papers.
– The points made about have always been particularly troublesome for working with [Top UK University] due to the[Top UK University] Innovations licensing arrangements but I think as that arrangement expires there’s recognition that companies can’t keep sinking massive grants into Universities unless they’re philanthropic without new creative commercial ways of working.
– Linked to the above two points one of the frustrations for industry is that a low TRL development that appears to be 80% of the commercial offer realised in a Uni can be achieved in 20% of the time but the other “20%” productisation to commercial fruition / TRL7 will be 800% of the industry partners production costs and associated time etc… This should be reflected in the engagement and IP position but isn’t really.
– Academia is only just recognising that it must adjust to collaborate or risk being out competed where “Quantum compute” or “fundamental battery tech”,etc ,etc research groups are appearing in bigger tech companies.
Caveat – my subjective view out of ignorance from the fringes: The EPSRC Industrial Strategy Challenge Fund and Prosperity Partnerships are a massive opportunity and yet the ISCF Waves that have appeared appear to have done so with limited industrial awareness, formal structure and engagement. So those that have been engaged have been at the table more likely through personal relationships, etc. So this needs more publicity and more formality… There also needs to be a clear understanding of Innovate UK, the Catapults’ and Research Councils’ roles.
I’m not sure I have a great answer to this but I think it’s an interesting question. In the distributed systems world academia plays an important role, but there is always a divide. Things that I think might be useful:
– Doing more to reach the audience in industry. The best example of this i’ve seen is https://blog.acolyer.org/.
– Partnering to study why things work well in practice rather than in theory. For example there is much the wider community can learn from the internal design decisions made by key open source components that run in the real world. So in my field the design decisions made building Kafka, Cassandra, Zookeeper, HBase could use further study which would be useful for the next iteration of technologies.
– Making it easier for industrial practitioners to play a role in academia. I know a few people that do this, but i’m not entirely sure how it works, but I feel it could be done more.
Finally some comments on twitter here: https://twitter.com/benstopford/status/917991118058459138
Delete Arbitrary Messages from a Kafka
Friday, October 6th, 2017
I’ve been asked a few times about how you can delete messages from a topic in Kafka. So for example, if you work for a company and you have a central Kafka instance, you might want to ensure that you can delete any arbitrary message due to say regulatory or data protection requirements or maybe simple in case something gets corrupted.
A potential trick to do this is to use a combination of (a) a compacted topic and (b) a custom partitioner (c) a pair of interceptors.
The process would follow:
- Use a producer interceptor to add a GUID to the end of the key before it is written.
- Use a custom partitioner to ignore the GUID for the purposes of partitioning
- Use a compacted topic so you can then delete any individual message you need via producer.send(key+GUID, null)
- Use a consumer interceptor to remove the GUID on read.
Two caveats: (1) Log compaction does not touch the most recent segment, so values will only be deleted once the first segment rolls. This essentially means it may take some time for the ‘delete’ to actually occur. (2) I haven’t tested this!
Slides Kafka Summit SF – Building Event-Driven Services with Stateful Streams
Monday, August 28th, 2017
Devoxx 2017 – Rethinking Services With Stateful Streams
Friday, May 12th, 2017
The Data Dichotomy
Wednesday, December 14th, 2016
A post about services and data, published on the Confluent site.
https://www.confluent.io/blog/data-dichotomy-rethinking-the-way-we-treat-data-and-services/
QCon Interview on Microservices and Stream Processing
Friday, February 19th, 2016
This is a transcript from an interview I did for QCon (delivered verbally):
QCon: What is your main focus right now at Confluent?
Ben: I work as an engineer in the Apache Kafka Core Team. I do some system architecture work too. At the moment, I am working on automatic data balancing within Kafka. Auto data balancing is basically expanding, contracting and balancing resources within the cluster as you add/remove machines or add some other kind of constraint or invariant. Basically, I’m working on making the cluster grow and shrink dynamically.
QCon: Is stream processing new?
Ben: Stream processing, as we know it, has really come from the background of batch analytics (around Hadoop) and that has kind of evolved into this stream processing thing as people needed to get things done faster.Although to be honest, stream processing has been around for 30 years in one form or another, but it has just always been quite niche. It’s only recently that it’s moved mainstream. That’s important because if you look at the stream processing technology from a decade ago, it was just a bit more specialist, less scalable, less available and less accessible (though, certainly not simple). Now that stream processing is more mainstream, it comes with a lot of quite powerful tooling and the ecosystem is just much bigger.
QCon: Why do you feel streaming data is an important consideration for Microservice architectures?
Ben: So you don’t see people talking about stream processing and Microservices together all that much. This is largely because they came from different places. But stream processing turns out to be pretty interesting from the Microservice perspective because there’s a bunch of overlap in the problems they need to solve as data scales out and business workflows cross service and data boundaries.
As you move from a monolithic application to a set of distributed services, you end up with much more complicated systems to plan and build (whether you like it or not). People typically have ReST for Request/Response, but most of the projects we see have moved their fact distribution to some sort of brokered approach, meaning they end up with some combination of request/response and event-based processing. So if ReST is at one side of the spectrum, then Kafka is at the other and the two end up being pretty complimentary. But there is actually a cool interplay between these two when you start thinking about it. Synchronous communication works well for a bunch of use cases, particularly GUIs or external services that are inherently RPC. Event-driven methods tend to work better for business processes, particularly as they get larger and more complex. This leads to patterns that end up looking a lot like event-driven architectures.
So when we actually build these things a bunch of problems pop up because no longer do we have a single shared database. We have no global bag of state in the sky to lean on. Sure, we have all the benefits of bounded contexts, nicely decoupled from the teams around them and this is pretty great for the most part. Database couplings have always been a bit troublesome and hard to manage. But now we hit all the pains of a distributed system and this means we end up having to be really careful about how we sew data together so we don’t screw it up along the way.
Relying on a persistent, distributed log helps with some of these problems. You can blend the good parts of shared statefulness and reliability without the tight centralised couplings that come with a shared database. That’s actually pretty useful from a microservices perspective because you can lean on the backing layer for a bunch of stuff around durability, availability, recovery, concurrent processing and the like.
But it isn’t just durability and history that helps. Services end up having to tackle a whole bunch of other problems that share similarities with stream processing systems. Scaling out, providing redundancy at a service level, dealing with sources that can come and go, where data may arrive on time or may be held up. Combining data from a bunch of different places. Quite often this ends up being solved by pushing everything into a database, inside the service, and querying it there, but that comes with a bunch of problems in its own right.
So a lot of the functions you end up building to do processing in these services, overlap with what stream processing engines do: join tables and streams from different places, create views that match your own domain model. Filter, aggregate, window these things further. Put this alongside a highly available distributed log and you start to get a pretty compelling toolset for building services that scale simply and efficiently.
QCon: What’s the core message of your talk?
Ben: So the core message is pretty simple. There’s a bunch of stuff going on over there, there’s a bunch of stuff going on over here. Some people are mashing this stuff together and some pretty interesting things are popping out. It’s about bringing these parts of industry together. So utilizing a distributed log as a backbone has some pretty cool side effects. Add a bit of stream processing into the mix and it all gets a little more interesting still.
So say you’re replacing a mainframe with a distributed service architecture, running on the cloud, you actually end up hitting a bunch of the same problems you hit in the analytic space as you try to get away from the Lambda Architecture.
The talk dives into some of these problems and tries to spell out a different way of approaching them from a services perspective, but using a stream processing toolset. Interacting with external sources, slicing and dicing data, dealing with windowing, dealing with exactly once processing, and not just from the point of view of web logs or social data. We’ll be thinking business systems, payment processing and the like.
The Benefits of “In-Memory” Data are Often Overstated
Sunday, January 3rd, 2016
There is an intuition we all share that memory is king. It’s fast, sexy and blows the socks off old school spinning disks or even new school SSDs. At it’s heart this is of course true. But when it comes to in-memory data technologies, alas it’s a lie. At least on balance.
The simple truth is this. The benefit of an in memory “database” (be it a db, distributed cache, data grid or something else) is two fold.
(1) It doesn’t have to use a data-structure that is optimised for disk. What that really means is you don’t need to read in pages like a database does, and you don’t have to serialise/deserialise between the database and your program. You just use whatever you domain model is in memory.
(2) You can load data into an in-memory database faster. The fastest disk backed databases top out bulk loads at around 100MB/s (~GbE network).
That’s it.There is nothing else. Reads will be no faster. There are many other downsides.
Don’t believe me? read on.
Lets consider why we use disk at all. To gain a degree of fault tolerance is common. We want to be able to pull the plug without fear of losing data. But if we have the data safely held elsewhere this isn’t such a big deal.
Disk is also useful for providing extra storage. Allowing us to ‘overflow’ our available memory. This can become painful, if we take the concept too far. The sluggish performance of an overladed PC that’s constantly paging memory to and from disk in an intuitive example, but this approach actually proves to be very successful in many data technologies, when the commonly used dataset fits largely in memory.
The operating system’s page cache is a key ingredient here. It’ll happily gobble up any available RAM, making many disk-backed solutions perform similarly to in-memory ones when there’s enough memory to play with. This applies to both reads and writes too, assuming the OS is left to page data to disk in its own time.
So this means the two approaches often perform similarly. Say we have two 128GB machines. On one we install an in-memory database. On the other we install a similar disk-backed database. We put 100GB of data into each of them. The disk-backed database will be reading data from memory most of the time. But it’ll also let you overflow beyond 128GB, pushing infrequently used data (which is common in most systems) onto disk so it doesn’t clutter the address space.
Now the tradeoff is a little subtler in reality. An in-memory database can guarantee comparatively fast random access. This gives good breadth for optimisation. On the other hand, the disk-backed database must use data structures optimised for the sequential approaches that magnetic (and to a slightly lesser extent SSD) based media require for good performance, even if the data is actually being served from memory.
So if the storage engine is something like a LSM tree there will be an associated overhead that the in-memory solution would not need to endure. This is undoubtedly significant, but we are still left wondering whether the benefit of this optimisation is really worth the downsides a of pure, in-memory solution.
Another subtlety relates to something we mentioned earlier. We may use disk for fault tolerance. A typical disk-backed database, like Postgres or Cassandra, uses disk in two different ways. The storage engine will use a file structure that is read-optimised in some way. In most cases an additional structure is used, generally termed a Write Ahead Log. This provides a fast way for logging data to a persistent media so the database can reply to clients in the knowledge that data is safe.
Now some in-memory databases neglect durability completely. Others provide durability through replication (a second replica exists on another machine using some clustering protocol). This later pattern has much value as it increases availability in failure scenarios. But this concern is really orthogonal. If you need a write ahead log use one, or use replicas. Whether your dataset is pinned entirely in memory, or can overflow to disk, is a separate concern.
A different reason to turn to a purely in-memory solution is to host a database in-process (i.e. in the program you are querying from). In this case the performance gain comes largely from the shared address space, lack of network IO, and maybe a lack of de/serialisation etc. This is valuable for applications which make use of local data processing. But all the arguments above still apply and disk overflow is again, often sensible.
So the key point is really that having disk around, as something to overflow into, is well worth the marginal tradeoff in performance. This is particularly true from an operational perspective. There is no hard ceiling, which means you can run closer to the limit without fear of failure. This makes disk-backed solution cheaper and less painful to run. The overall cost of write amplification (the additional storage overhead associated with each record) is often underestimated** meaning we often hit the memory wall sooner than we’d like. Moreover the reality of most projects is that a small fraction of the data held is used frequently, so paying the price of holding that in RAM can become a burden as datasets grow… and datasets always grow!
There is also reason to urge caution though. The disk-is-slow intuition is absolutely correct. Push your disk-backed dataset to the point where the disk is being used for frequent random access and performance is going to end up falling off a very steep cliff. The point is simply that, for many use cases, there’s likely more wiggle room than you may think.
So memory optimised is good. Memory optimised is fast. But the downsides of the hard limit imposed by pure in-memory solutions is often not worth the operational burden, especially when disk backed solutions, provided ample memory to use for caching, perform equally well for all but the most specialised, data intensive use cases.
** When I worked with distributed caches a write amplification of x6 was typical in real world systems. This was made from a number of factors: Primary and replica copies, JVM overhead, data skew across the cluster, overhead of Java objects representations, indexes.
Elements of Scale: Composing and Scaling Data Platforms
Tuesday, April 28th, 2015
This post is the transcript from a talk, of the same name, given at Progscon & JAX Finance 2015.
There is a video also.
As software engineers we are inevitably affected by the tools we surround ourselves with. Languages, frameworks, even processes all act to shape the software we build.
Likewise databases, which have trodden a very specific path, inevitably affect the way we treat mutability and share state in our applications.
Over the last decade we’ve explored what the world might look like had we taken a different path. Small open source projects try out different ideas. These grow. They are composed with others. The platforms that result utilise suites of tools, with each component often leveraging some fundamental hardware or systemic efficiency. The result, platforms that solve problems too unwieldy or too specific to work within any single tool.
So today’s data platforms range greatly in complexity. From simple caching layers or polyglotic persistence right through to wholly integrated data pipelines. There are many paths. They go to many different places. In some of these places at least, nice things are found.
So the aim for this talk is to explain how and why some of these popular approaches work. We’ll do this by first considering the building blocks from which they are composed. These are the intuitions we’ll need to pull together the bigger stuff later on.
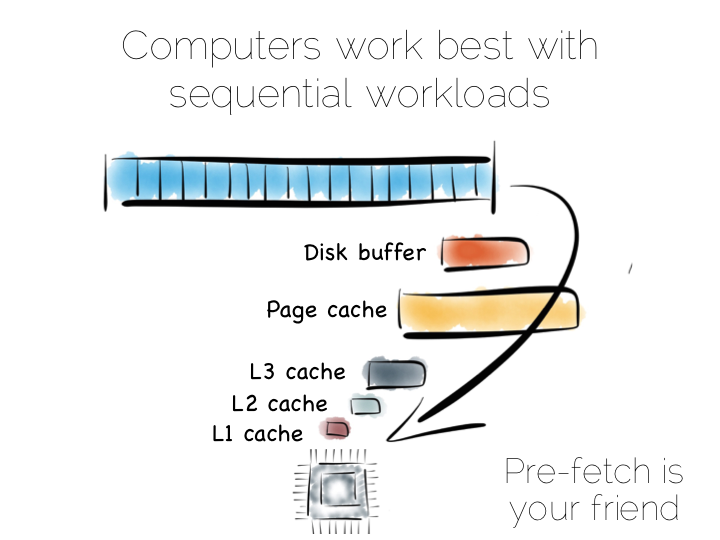
In a somewhat abstract sense, when we’re dealing with data, we’re really just arranging locality. Locality to the CPU. Locality to the other data we need. Accessing data sequentially is an important component of this. Computers are just good at sequential operations. Sequential operations can be predicted.
If you’re taking data from disk sequentially it’ll be pre-fetched into the disk buffer, the page cache and the different levels of CPU caching. This has a significant effect on performance. But it does little to help the addressing of data at random, be it in main memory, on disk or over the network. In fact pre-fetching actually hinders random workloads as the various caches and frontside bus fill with data which is unlikely to be used.
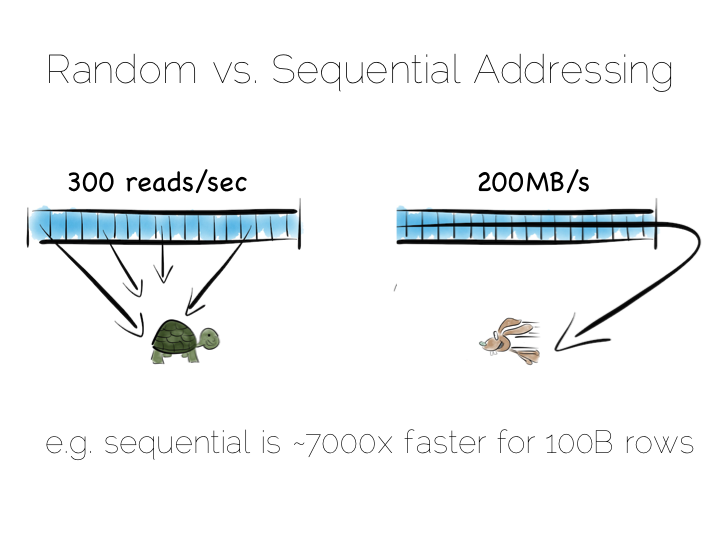
So whilst disk is somewhat renowned for its slow performance, main memory is often assumed to simply be fast. This is not as ubiquitously true as people often think. There are one to two orders of magnitude between random and sequential main memory workloads. Use a language that manages memory for you and things generally get a whole lot worse.
Streaming data sequentially from disk can actually outperform randomly addressed main memory. So disk may not always be quite the tortoise we think it is, at least not if we can arrange sequential access. SSD’s, particularly those that utilise PCIe, further complicate the picture as they demonstrate different tradeoffs, but the caching benefits of the two access patterns remain, regardless.
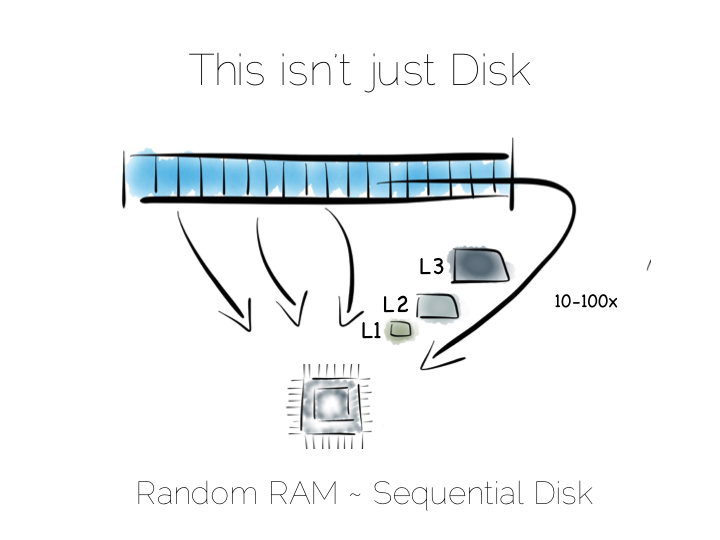
So lets imagine, as a simple thought experiment, that we want to create a very simple database. We’ll start with the basics: a file.
We want to keep writes and reads sequential, as it works well with the hardware. We can append writes to the end of the file efficiently. We can read by scanning the the file in its entirety. Any processing we wish to do can happen as the data streams through the CPU. We might filter, aggregate or even do something more complex. The world is our oyster!
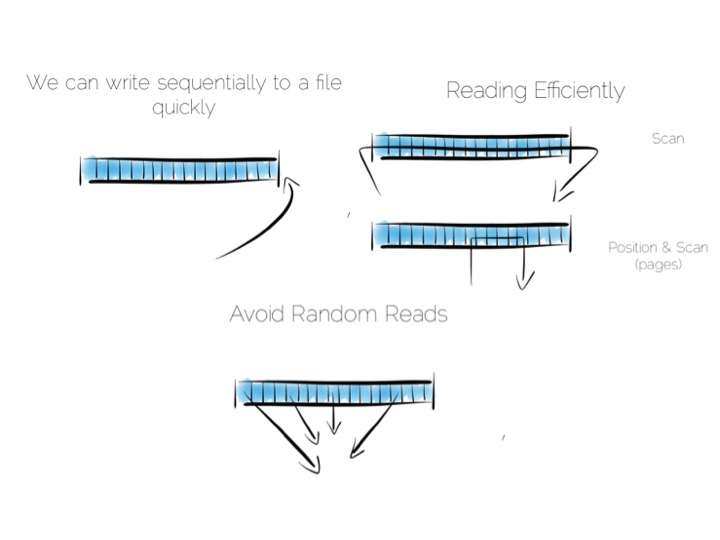
So what about data that changes, updates etc?
We have a couple of options. We could update the value in place. We’d need to use fixed width fields for this, but that’s ok for our little thought experiment. But update in place would mean random IO. We know that’s not good for performance.
Alternatively we could just append updates to the end of the file and deal with the superseded values when we read it back.
So we have our first tradeoff. Append to a ‘journal’ or ‘log’, and reap the benefits of sequential access. Alternatively if we use update in place we’ll be back to 300 or so writes per second, assuming we actually flush through to the underlying media.
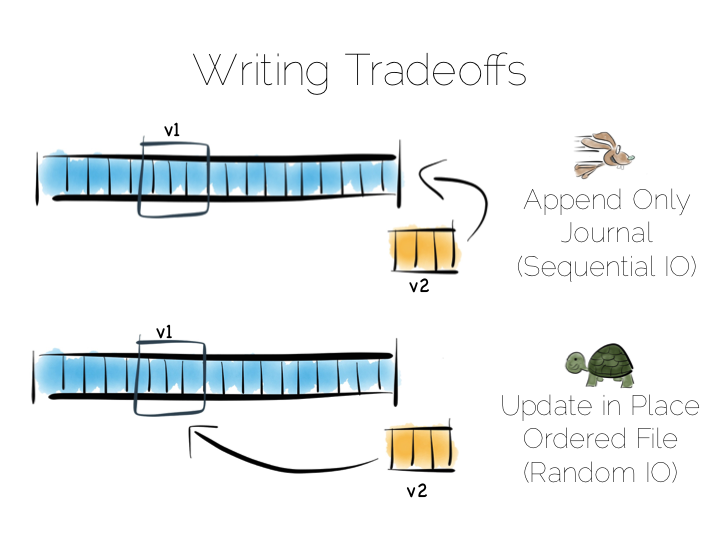
Now in practice of course reading the file, in its entirety, can be pretty slow. We’ll only need to get into GB’s of data and the fastest disks will take seconds. This is what a database does when it ends up table scanning.
Also we often want something more specific, say customers named “bob”, so scanning the whole file would be overkill. We need an index.
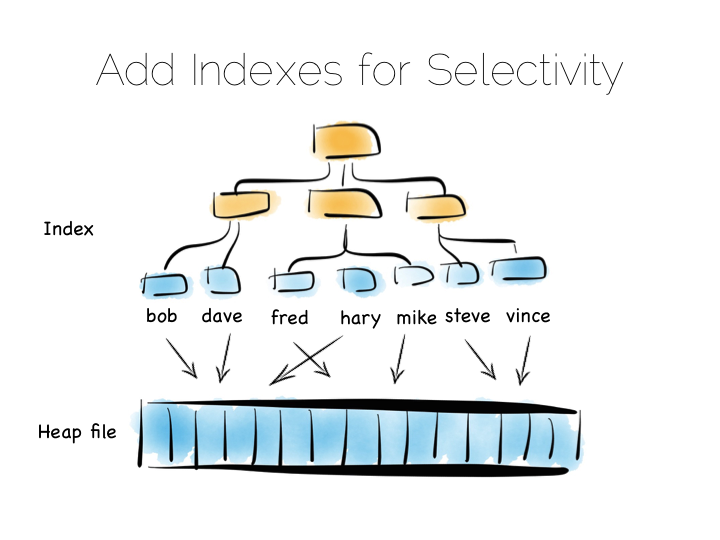
Now there are lots of different types of indexes we could use. The simplest would be an ordered array of fixed-width values, in this case customer names, held with the corresponding offsets in the heap file. The ordered array could be searched with binary search. We could also of course use some form of tree, bitmap index, hash index, term index etc. Here we’re picturing a tree.
The thing with indexes like this is that they impose an overarching structure. The values are deliberately ordered so we can access them quickly when we want to do a read. The problem with the overarching structure is that it necessitates random writes as data flows in. So our wonderful, write optimised, append only file must be augmented by writes that scatter-gun the filesystem. This is going to slow us down.
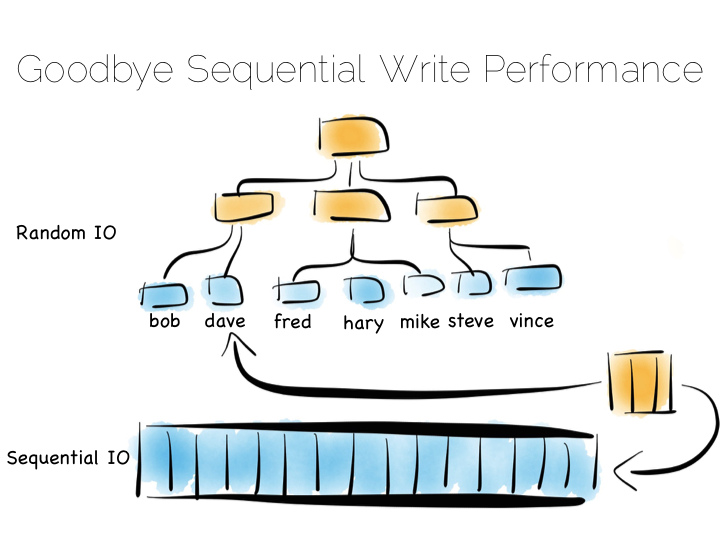
Anyone who has put lots of indexes on a database table will be familiar with this problem. If we are using a regular rotating hard drive, we might run 1,000s of times slower if we maintain disk integrity of an index in this way.
Luckily there are a few ways around this problem. Here we are going to discuss three. These represent three extremes, and they are in truth simplifications of the real world, but the concepts are useful when we consider larger compositions.
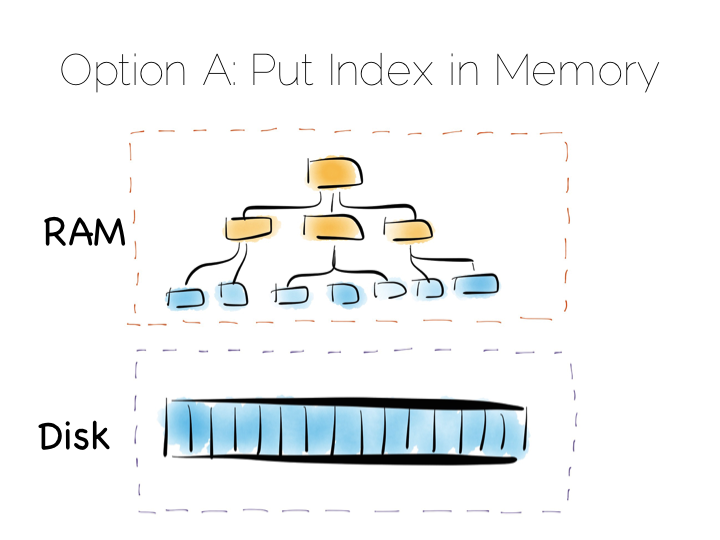
Our first option is simply to place the index in main memory. This will compartmentalise the problem of random writes to RAM. The heap file stays on disk.
This is a simple and effective solution to our random writes problem. It is also one used by many real databases. MongoDB, Cassandra, Riak and many others use this type of optimisation. Often memory mapped files are used.
However, this strategy breaks down if we have far more data than we have main memory. This is particularly noticeable where there are lots of small objects. Our index would get very large. Thus our storage becomes bounded by the amount of main memory we have available. For many tasks this is fine, but if we have very large quantities of data this can be a burden.
A popular solution is to move away from having a single ‘overarching’ index. Instead we use a collection of smaller ones.
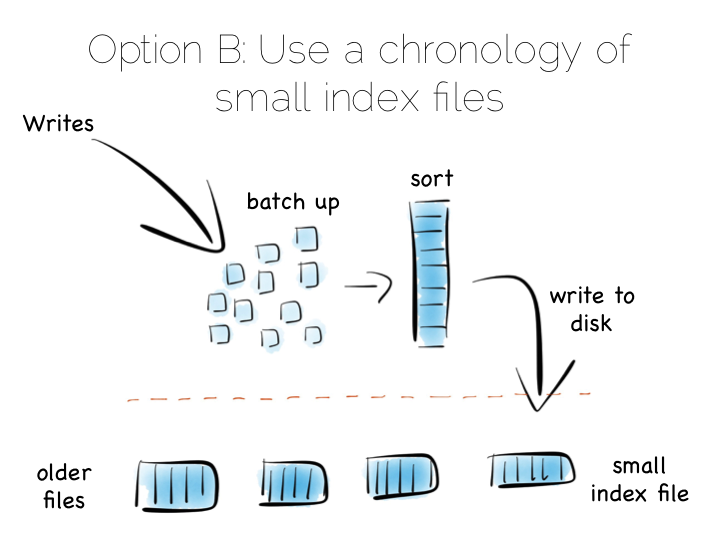
This is a simple idea. We batch up writes in main memory, as they come in. Once we have sufficient – say a few MB’s – we sort them and write them to disk as an individual mini-index. What we end up with is a chronology of small, immutable index files.
So what was the point of doing that? Our set of immutable files can be streamed sequentially. This brings us back to a world of fast writes, without us needing to keep the whole index in memory. Nice!
Of course there is a downside to this approach too. When we read, we have to consult the many small indexes individually. So all we have really done is shift the problem of RandomIO from writes onto reads. However this turns out to be a pretty good tradeoff in many cases. It’s easier to optimise random reads than it is to optimise random writes.
Keeping a small meta-index in memory or using a Bloom Filter provides a low-memory way of evaluating whether individual index files need to be consulted during a read operation. This gives us almost the same read performance as we’d get with a single overarching index whilst retaining fast, sequential writes.
In reality we will need to purge orphaned updates occasionally too, but that can be done with nice sequential reads and writes.
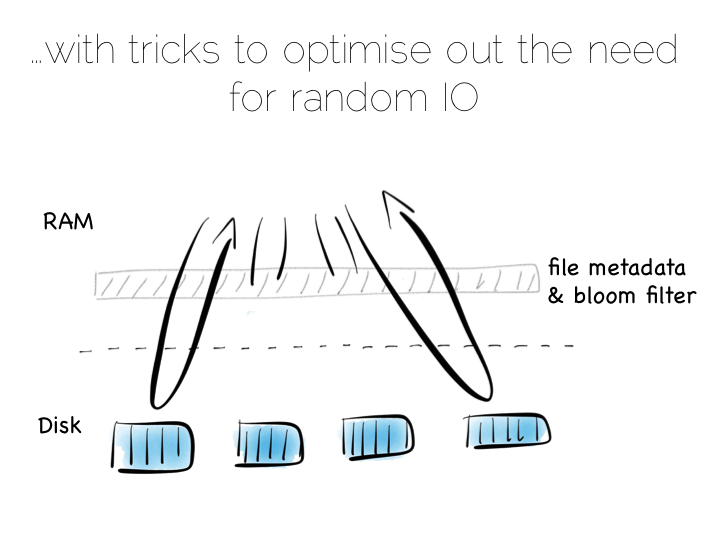
What we have created is termed a Log Structured Merge Tree. A storage approach used in a lot of big data tools such as HBase, Cassandra, Google’s BigTable and many others. It balances write and read performance with comparatively small memory overhead.
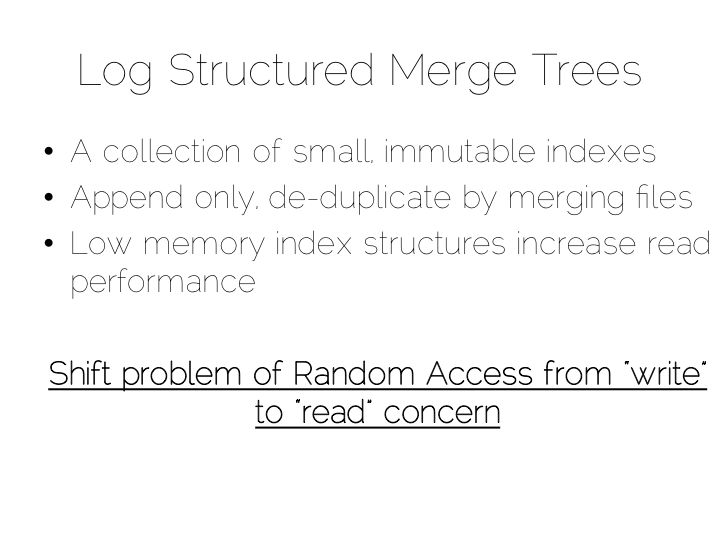
So we can get around the ‘random-write penalty’ by storing our indexes in memory or, alternatively, using a write-optimised index structure like LSM. There is a third approach though. Pure brute force.
Think back to our original example of the file. We could read it in its entirety. This gave us many options in terms of how we go about processing the data within it. The brute force approach is simply to hold data by column rather than by row. This approach is termed Columnar or Column Oriented.
(It should be noted that there is an unfortunate nomenclature clash between true column stores and those that follow the Big Table pattern. Whilst they share some similarities, in practice they are quite different. It is wise to consider them as different things.)
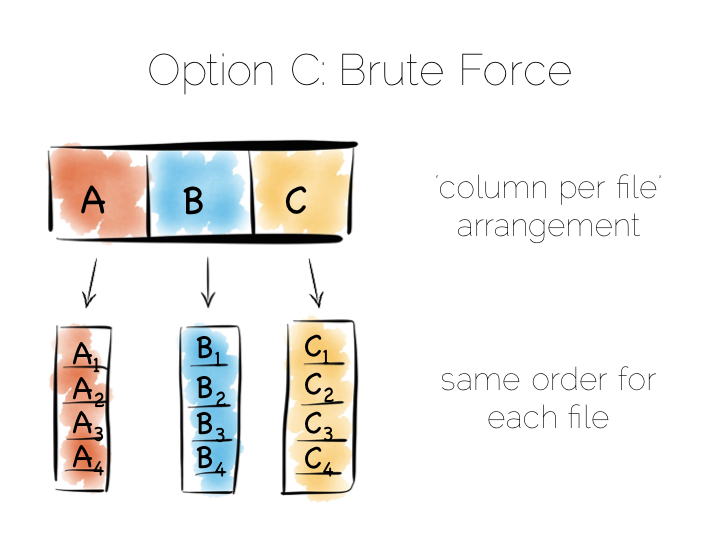
Column Orientation is another simple idea. Instead of storing data as a set of rows, appended to a single file, we split each row by column. We then store each column in a separate file. When we read we only read the columns we need.
We keep the order of the files the same, so row N has the same position (offset) in each column file. This is important because we will need to read multiple columns to service a single query, all at the same time. This means ‘joining’ columns on the fly. If the columns are in the same order we can do this in a tight loop which is very cache- and cpu-efficient. Many implementations make heavy use of vectorisation to further optimise throughput for simple join and filter operations.
Writes can leverage the benefits of being append-only. The downside is that we now have many files to update, one for every column in every individual write to the database. The most common solution to this is to batch writes in a similar way to the one used in the LSM approach above. Many columnar databases also impose an overall order to the table as a whole to increase their read performance for one chosen key.
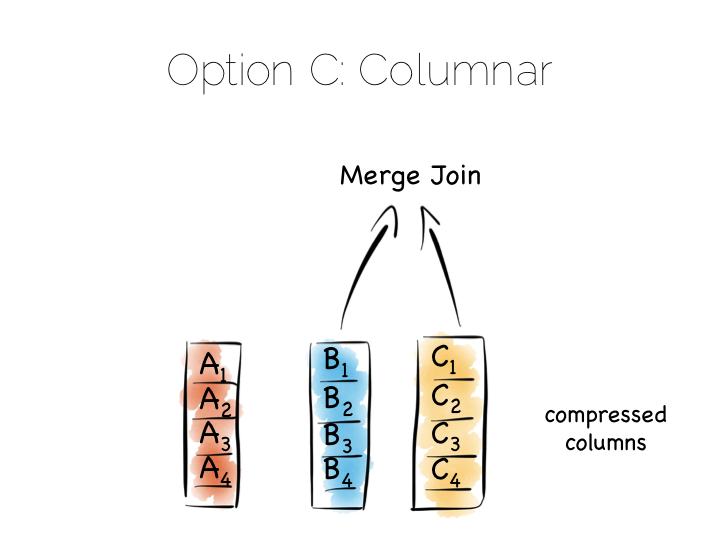
By splitting data by column we significantly reduce the amount of data that needs to be brought from disk, so long as our query operates on a subset of all columns.
In addition to this, data in a single column generally compresses well. We can take advantage of the data type of the column to do this, if we have knowledge of it. This means we can often use efficient, low cost encodings such as run-length, delta, bit-packed etc. For some encodings predicates can be used directly on the compressed stream too.
The result is a brute force approach that will work particularly well for operations that require large scans. Aggregate functions like average, max, min, group by etc are typical of this.
This is very different to using the ‘heap file & index’ approach we covered earlier. A good way to understand this is to ask yourself: what is the difference between a columnar approach like this vs a ‘heap & index’ where indexes are added to every field?
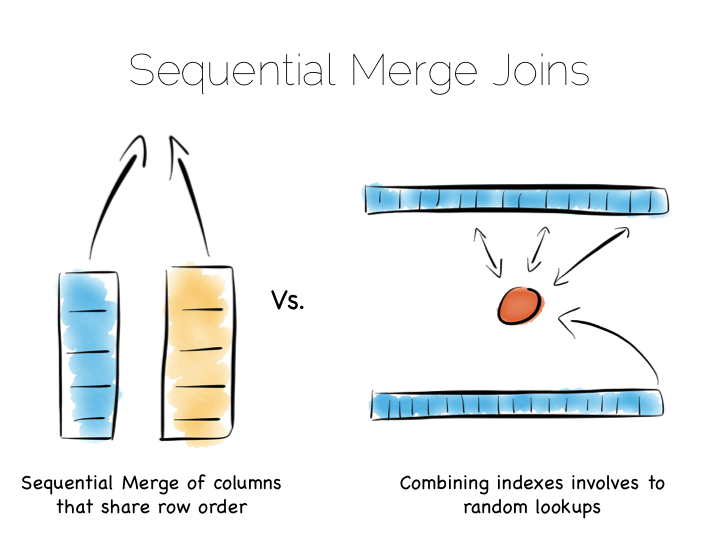
The answer to this lies in the ordering of the index files. BTrees etc will be ordered by the fields they index. Joining the data in two indexes involves a streaming operation on one side, but on the other side the index lookups have to read random positions in the second index. This is generally less efficient than joining two indexes (columns) that retain the same ordering. Again we’re leveraging sequential access.
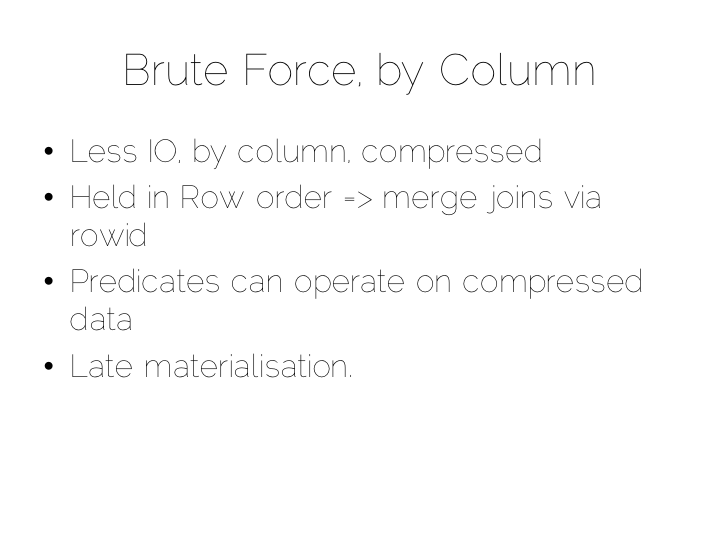
So many of the best technologies which we may want to use as components in a data platform will leverage one of these core efficiencies to excel for a certain set of workloads.
Storing indexes in memory, over a heap file, is favoured by many NoSQL stores such as Riak, Couchbase or MongoDB as well as some relational databases. It’s a simple model that works well.
Tools designed to work with larger data sets tend to take the LSM approach. This gives them fast ingestion as well as good read performance using disk based structures. HBase, Cassandra, RocksDB, LevelDB and even Mongo now support this approach.
Column-per-file engines are used heavily in MPP databases like Redshift or Vertica as well as in the Hadoop stack using Parquet. These are engines for data crunching problems that require large traversals. Aggregation is the home ground for these tools.
Other products like Kafka apply the use of a simple, hardware efficient contract to messaging. Messaging, at its simplest, is just appending to a file, or reading from a predefined offset. You read messages from an offset. You go away. You come back. You read from the offset you previously finished at. All nice sequential IO.
This is different to most message oriented middleware. Specifications like JMS and AMQP require the addition of indexes like the ones discussed above, to manage selectors and session information. This means they often end up performing more like a database than a file. Jim Gray made this point famously back in his 1995 publication Queue’s are Databases.
So all these approaches favour one tradeoff or other, often keeping things simple, and hardware sympathetic, as a means of scaling.
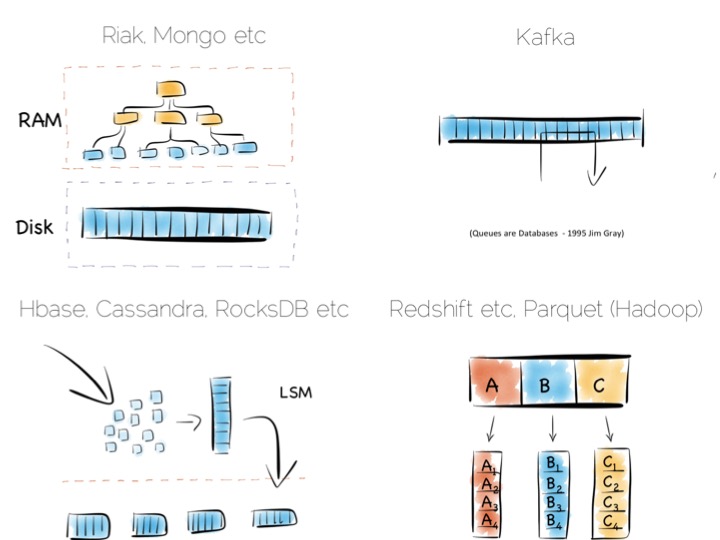
So we’ve covered some of the core approaches to storage engines. In truth we made some simplifications. The real world is a little more complex. But the concepts are useful nonetheless.
Scaling a data platform is more than just storage engines though. We need to consider parallelism.
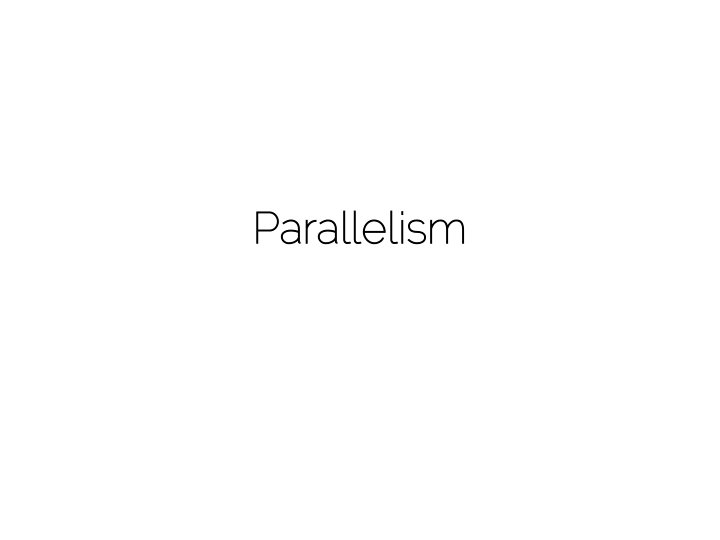
When distributing data over many machines we have two core primitives to play with: partitioning and replication. Partitioning, sometimes called sharding, works well both for random access and brute force workloads.
If a hash-based partitioning model is used the data will be spread across a number of machines using a well-known hash function. This is similar to the way a hash table works, with each bucket being held on a different machine.
The result is that any value can be read by going directly to the machine that contains the data, via the hash function. This pattern is wonderfully scalable and is the only pattern that shows linear scalability as the number of client requests increases. Requests are isolated to a single machine. Each one will be served by just a single machine in the cluster.
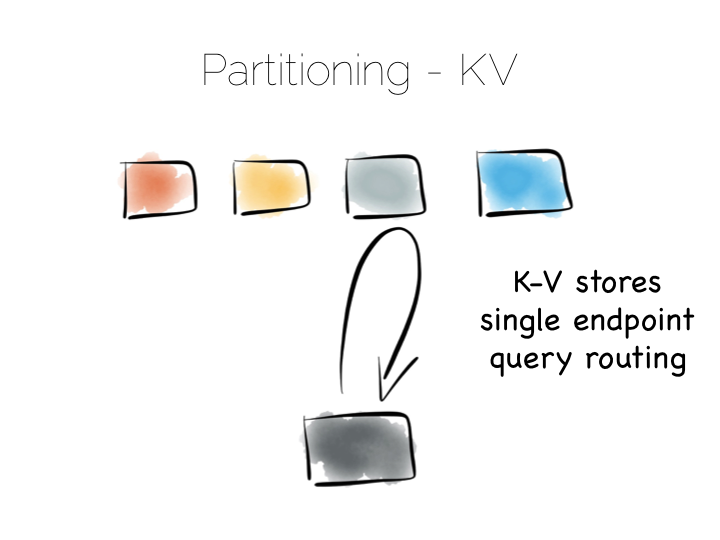
We can also use partitioning to provide parallelism over batch computations, for example aggregate functions or more complex algorithms such as those we might use for clustering or machine learning. The key difference is that we exercise all machines at the same time, in a broadcast manner. This allows us to solve a large computational problem in a much shorter time, using a divide and conquer approach.
Batch systems work well for large problems, but provide little concurrency as they tend to exhaust the resources on the cluster when they execute.
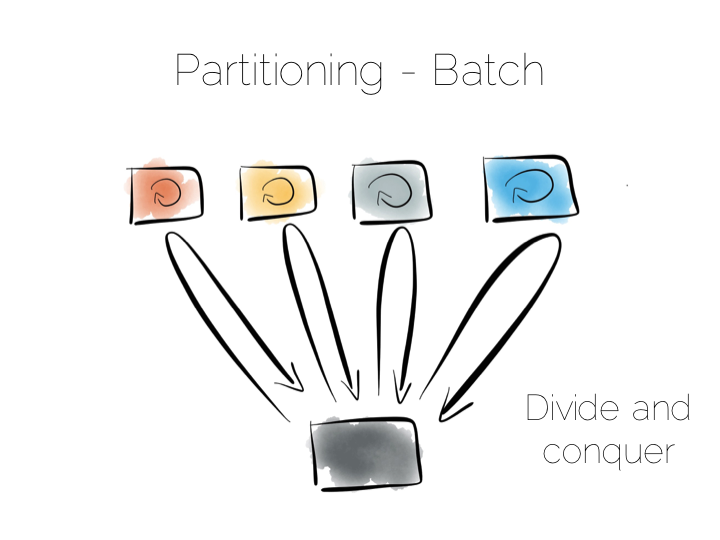
So the two extremes are pretty simple: Directed access at one end. Broadcast, divide and conquer at the other. Where we need to be careful is in the middle ground that lies between the two. A good example of this is the use of secondary indexes in NoSQL stores that span many machines.
A secondary index is an index that isn’t on the primary key. This means the data will not be partitioned by the values in the index. Directed routing via a hash function is no longer an option. We have to broadcast requests to all machines. This limits concurrency. Every node must be involved in every query.
For this reason many key value stores have resisted the temptation to add secondary indexes, despite their obvious use. HBase and Voldemort are examples of this. But many others do expose them, MongoDB, Cassandra, Riak etc. This is good as secondary indexes are useful. But it’s important to understand the effect they will have on the overall concurrency of the system.
The route out of this concurrency bottleneck is replication. You’ll probably be familiar with replication either from using async slave databases or from replicated NoSQL stores like Mongo or Cassandra.
In practice replicas can be invisible (used only for recovery), read only (adding concurrency) or read-write (adding availability under network partitions). Which of these you choose will trade off against the consistency of the system. This is simply the application of CAP theorem (although cap theorem also may not be as simple as you think).
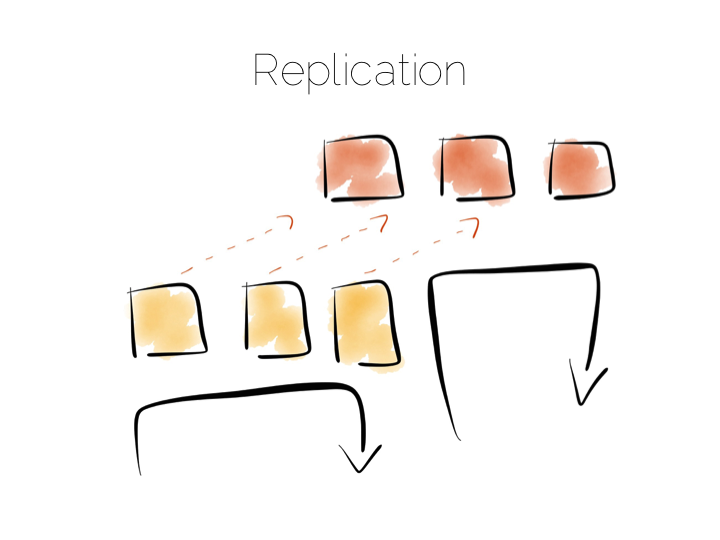
This tradeoff with consistency* brings us to an important question. When does consistency matter?
Consistency is expensive. In the database world ACID is guaranteed by linearisabilty. This is essentially ensuring that all operations appear to occur in sequential order. It turns out to be a pretty expensive thing. In fact it’s prohibitive enough that many databases don’t offer it as an isolation level at all. Those that do, rarely set it as the default.
Suffice to say that if you apply strong consistency to a system that does distributed writes you’ll likely end up in tortoise territory.
(* note the term consistency has two common usages. The C in ACID and the C in CAP. They are unfortunately not the same. I’m using the CAP definition: all nodes see the same data at the same time)
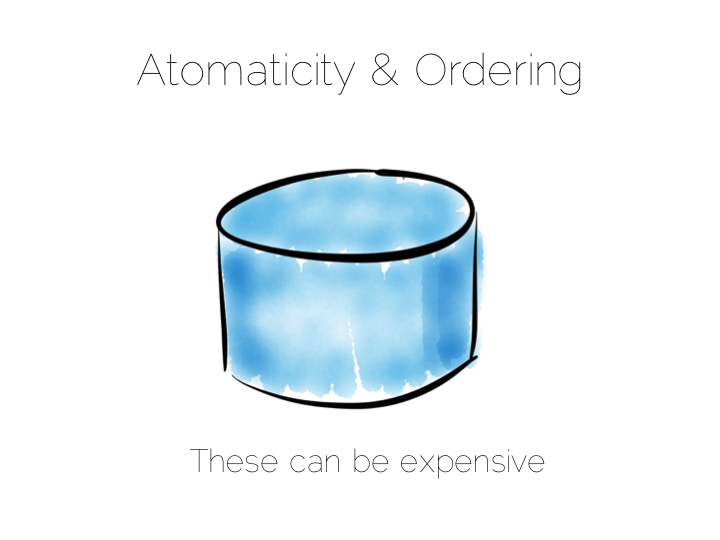
The solution to this consistency problem is simple. Avoid it. If you can’t avoid it isolate it to as few writers and as few machines as possible.
Avoiding consistency issues is often quite easy, particularly if your data is an immutable stream of facts. A set of web logs is a good example. They have no consistency concerns as they are just facts that never change.
There are other use cases which do necessitate consistency though. Transferring money between accounts is an oft used example. Non-commutative actions such as applying discount codes is another.
But often things that appear to need consistency, in a traditional sense, may not. For example if an action can be changed from a mutation to a new set of associated facts we can avoid mutable state. Consider marking a transaction as being potentially fraudulent. We could update it directly with the new field. Alternatively we could simply use a separate stream of facts that links back to the original transaction.
So in a data platform it’s useful to either remove the consistency requirement altogether, or at least isolate it. One way to isolate is to use the single writer principal, this gets you some of the way. Datomic is a good example of this. Another is to physically isolate the consistency requirement by splitting mutable and immutable worlds.
Approaches like Bloom/CALM extend this idea further by embracing the concept of disorder by default, imposing order only when necessary.
So those were some of the fundamental tradeoffs we need to consider. Now how to we pull these things together to build a data platform?
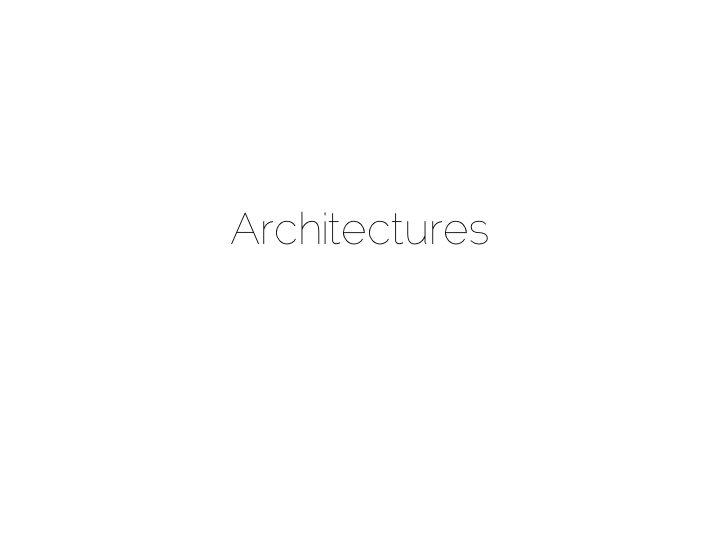
A typical application architecture might look something like the below. We have a set of processes which write data to a database and read it back again. This is fine for many simple workloads. Many successful applications have been built with this pattern. But we know it works less well as throughput grows. In the application space this is a problem we might tackle with message-passing, actors, load balancing etc.
The other problem is this approach treats the database as a black box. Databases are clever software. They provide a huge wealth of features. But they provide few mechanisms for scaling out of an ACID world. This is a good thing in many ways. We default to safety. But it can become an annoyance when scaling is inhibited by general guarantees which may be overkill for the requirements we have.
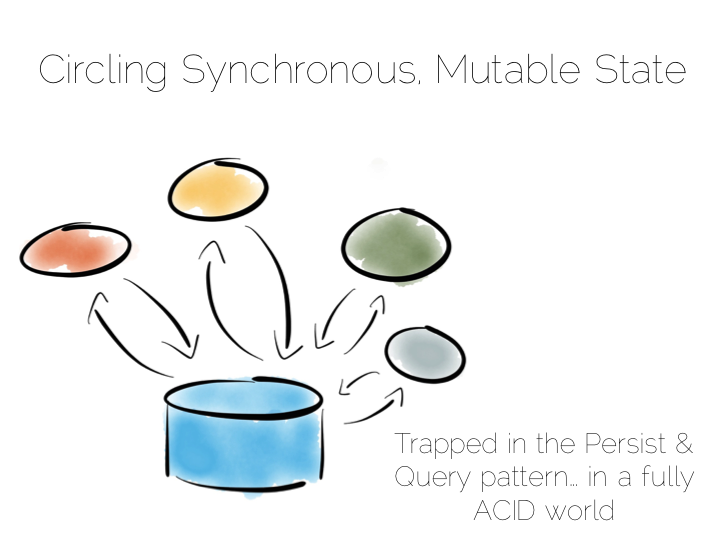
The simplest route out of this is CQRS (Command Query Responsibility Segregation).
Another very simple idea. We separate read and write workloads. Writes go into something write-optimised. Something closer to a simple journal file. Reads come from something read-optimised. There are many ways to do this, be it tools like Goldengate for relational technologies or products that integrate replication internally such as Replica Sets in MongoDB.
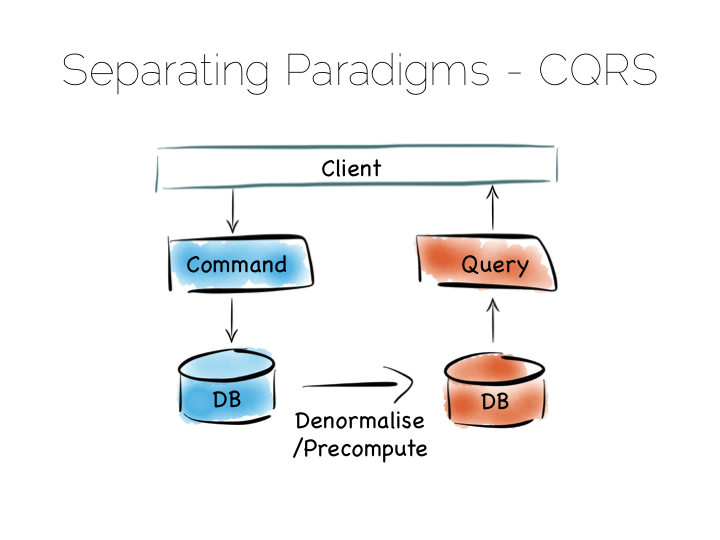
Many databases do something like this under the hood. Druid is a nice example. Druid is an open source, distributed, time-series, columnar analytics engine. Columnar storage works best if we input data in large blocks, as the data must be spread across many files. To get good write performance Druid stores recent data in a write optimised store. This is gradually ported over to the read optimised store over time.
When Druid is queried the query routes to both the write optimised and read optimised components. The results are combined (‘reduced’) and returned to the user. Druid uses time, marked on each record, to determine ordering.
Composite approaches like this provide the benefits of CQRS behind a single abstraction.
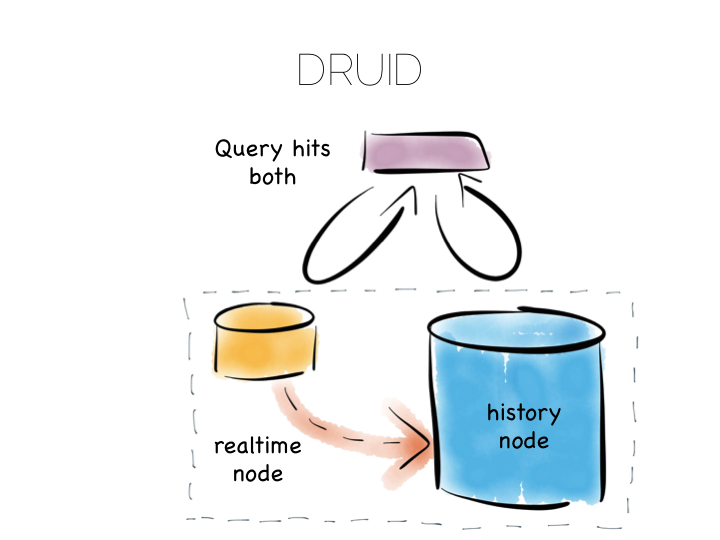
Another similar approach is to use an Operational/Analytic Bridge. Read- and write-optimised views are separated using an event stream. The stream of state is retained indefinitely, so that the async views can be recomposed and augmented at a later date by replaying.
So the front section provides for synchronous reads and writes. This can be as simple as immediately reading data that was written or as complex as supporting ACID transactions.
The back end leverages asynchronicity, and the advantages of immutable state, to scale offline processing through replication, denormalisation or even completely different storage engines. The messaging-bridge, along with joining the two, allows applications to listen to the data flowing through the platform.
As a pattern this is well suited to mid-sized deployments where there is at least a partial, unavoidable requirement for a mutable view.
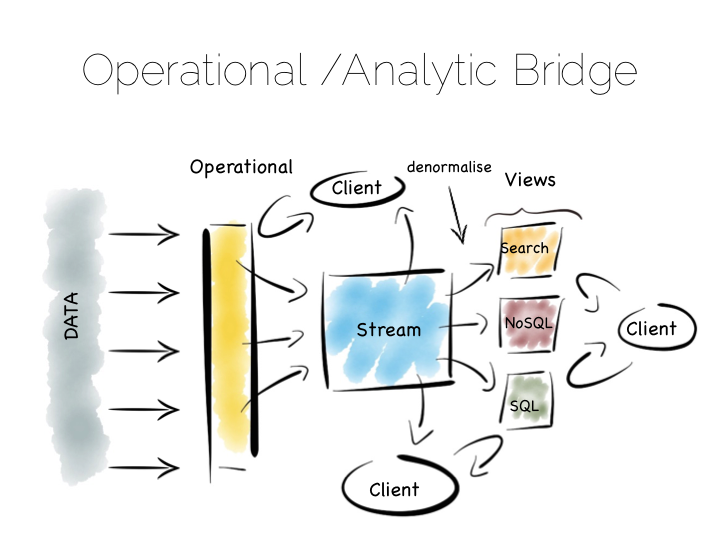
If we are designing for an immutable world, it’s easier to embrace larger data sets and more complex analytics. The batch pipeline, one almost ubiquitously implemented with the Hadoop stack, is typical of this.
The beauty of the Hadoop stack comes from it’s plethora of tools. Whether you want fast read-write access, cheap storage, batch processing, high throughput messaging or tools for extracting, processing and analysing data, the Hadoop ecosystem has it all.
The batch pipeline architecture pulls data from pretty much any source, push or pull. Ingests it into HDFS then processes it to provide increasingly optimised versions of the original data. Data might be enriched, cleansed, denormalised, aggregated, moved to a read optimised format such as Parquet or loaded into a serving layer or data mart. Data can be queried and processed throughout this process.
This architecture works well for immutable data, ingested and processed in large volume. Think 100’s of TBs (although size alone isn’t a great metric). The evolution of this architecture will be slow though. Straight-through timings are often measured in hours.
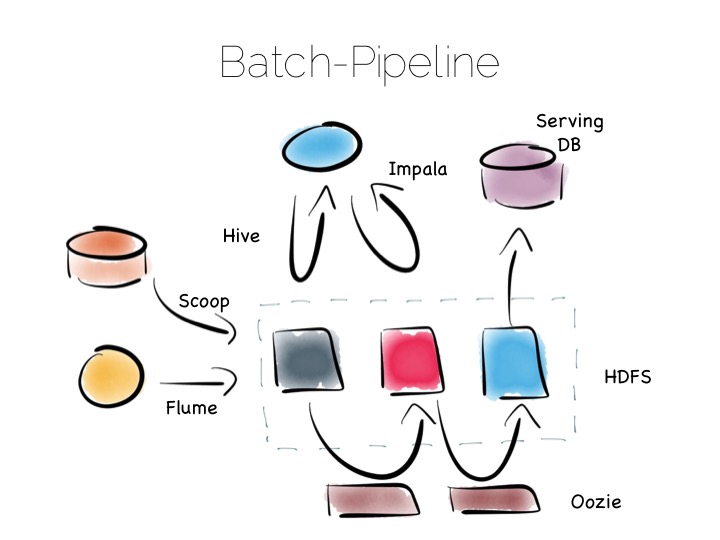
The problem with the Batch Pipeline is that we often don’t want to wait hours to get a result. A common solution is to add a streaming layer aside it. This is sometimes referred to as the Lambda Architecture.
The Lambda Architecture retains a batch pipeline, like the one above, but it circumvents it with a fast streaming layer. It’s a bit like building a bypass around a busy town. The streaming layer typically uses a streaming processing tool such as Storm or Samza.
The key insight of the Lambda Architecture is that we’re often happy to have an approximate answer quickly, but we would like an accurate answer in the end.
So the streaming layer bypasses the batch layer providing the best answers it can within a streaming window. These are written to a serving layer. Later the batch pipeline computes an accurate data and overwrites the approximation.
This is a clever way to balance accuracy with responsiveness. Some implementations of this pattern suffer if the two branches end up being dual coded in stream and batch layers. But it is often possible to simply abstract this logic into common libraries that can be reused, particularly as much of this processing is often written in external libraries such as Python or R anyway. Alternatively systems like Spark provide both stream and batch functionality in one system (although the streams in Spark are really micro-batches).
So this pattern again suits high volume data platforms, say in the 100TB range, that want to combine streams with existing, rich, batch based analytic function.
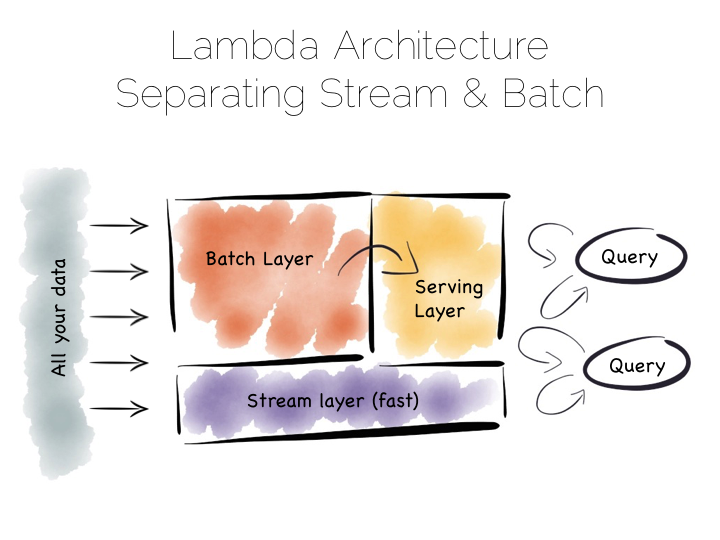
There is another approach to this problem of slow data pipelines. It’s sometimes termed the Kappa architecture. I actually thought this name was ‘tongue in cheek’ but I’m now not so sure. Whichever it is, I’m going to use the term Stream Data Platform, which is a term in use also.
Stream Data Platform’s flip the batch pattern on its head. Rather than storing data in HDFS, and refining it with incremental batch jobs, the data is stored in a scale out messaging system, or log, such as Kafka. This becomes the system of record and the stream of data is processed in real time to create a set of tertiary views, indexes, serving layers or data marts.
This is broadly similar to the streaming layer of the Lambda architecture but with the batch layer removed. Obviously the requirement for this is that the messaging layer can store and vend very large volumes of data and there is a sufficiently powerful stream processor to handle the processing.
There is no free lunch so, for hard problems, Stream Data Platform’s will likely run no faster than an equivalent batch system, but switching the default approach from ‘store and process’ to ‘stream and process’ can provide greater opportunity for faster results.
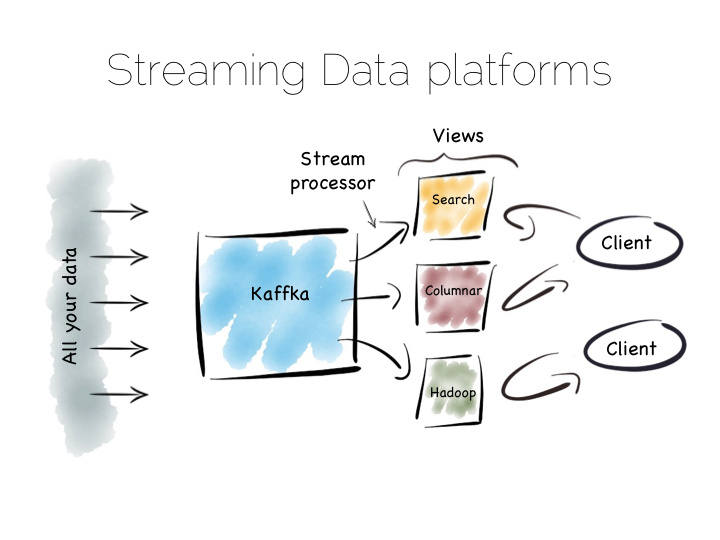
Finally, the Stream Data Platform approach can be applied to the problem of ‘application integration’. This is a thorny and difficult problem that has seen focus from big vendors such as Informatica, Tibco and Oracle for many years. For the most part results have been beneficial, but not transformative. Application integration remains a topic looking for a real workable solution.
Stream Data Platform’s provide an interesting potential solution to this problem. They take many of the benefits of an O/A bridge – the variety of asynchronous storage formats and ability to recreate views – but leave the consistency requirement isolated in, often existing sources:
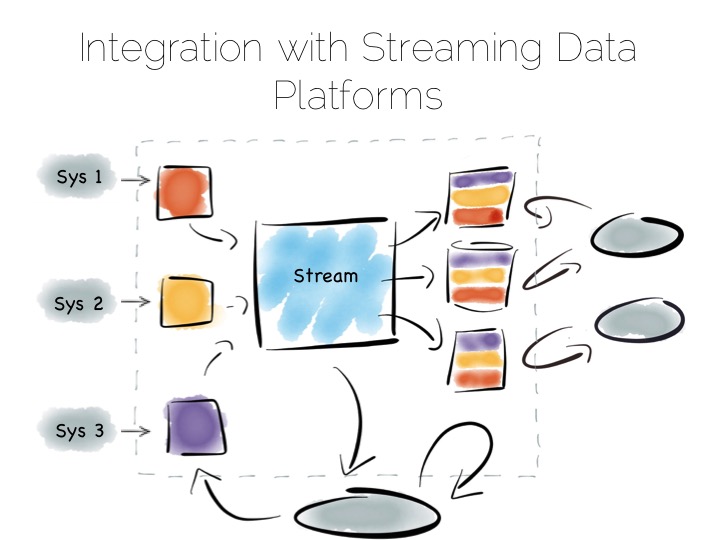
With the system of record being a log it’s easy to enforce immutability. Products like Kafka can retain enough volume and throughput, internally, to be used as a historic record. This means recovery can be a process of replaying and regenerating state, rather than constantly checkpointing.
Similarly styled approaches have been taken before in a number of large institutions with tools such as Goldengate, porting data to enterprise data warehouses or more recently data lakes. They were often thwarted by a lack of throughput in the replication layer and the complexity of managing changing schemas. It seems unlikely the first problem will continue. As for the later problem though, the jury is still out.
~
So we started with locality. With sequential addressing for both reads and writes. This dominates the tradeoffs inside the components we use. We looked at scaling these components out, leveraging primitives for both sharding and replication. Finally we rebranded consistency as a problem we should isolate in the platforms we build.
But data platforms themselves are really about balancing the sweet-spots of these individual components within a single, holistic form. Incrementally restructuring. Migrating the write-optimised to the read-optimised. Moving from the constraints of consistency to the open plains of streamed, asynchronous, immutable state.
This must be done with a few things in mind. Schemas are one. Time, the peril of the distributed, asynchronous world, is another. But these problems are manageable if carefully addressed. Certainly the future is likely to include more of these things, particularly as tooling, innovated in the big data space, percolates into platforms that address broader problems, both old and new.
~
THINGS WE LIKE
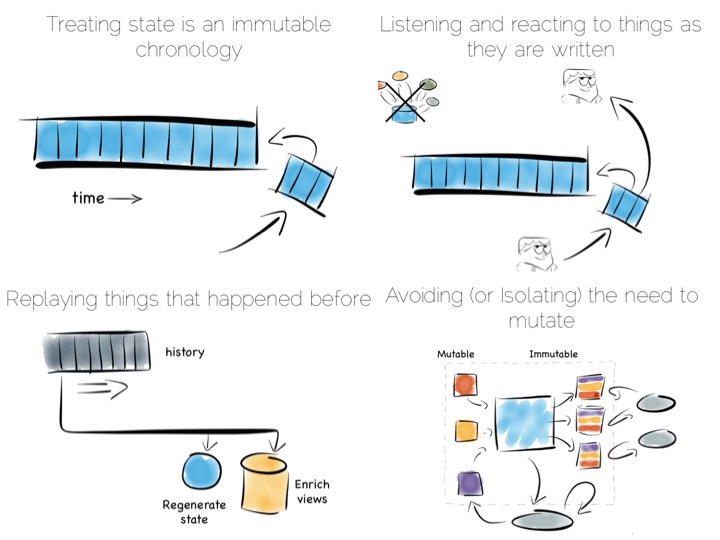
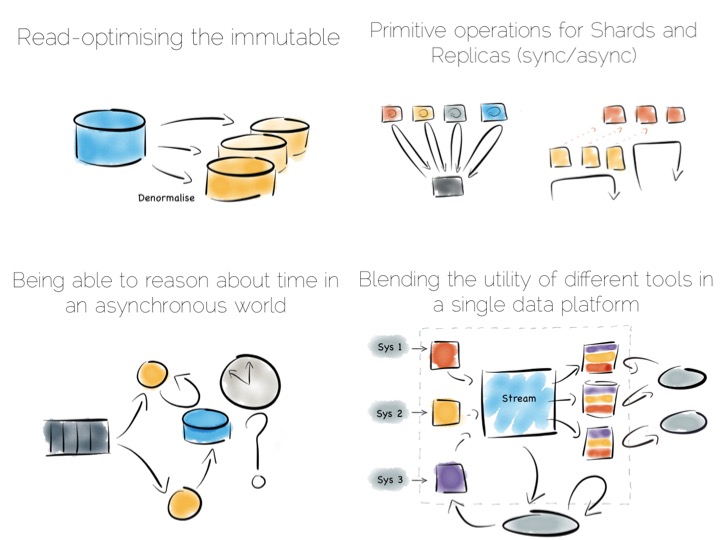
Upside Down Databases: Bridging the Operational and Analytic Worlds with Streams
Tuesday, April 7th, 2015
Remember the days when people would write entire applications, embedded inside a database? It seems a bit crazy now when you think about it. Imagine writing an entire application in SQL. I worked on a beast like that, very briefly, in the late 1990s. It had a few shell scripts but everything else was SQL. Everything. Suffice to say it wasn’t much fun – you can probably imagine – but there was a slightly perverse simplicity to the whole thing.
So Martin Kleppmann did a talk recently around the idea of turning databases inside out. I like this idea. It’s a nice way to frame a problem that has lurked unresolved for years. To paraphrase somewhat… databases do very cool stuff: caching, indexes, replication, materialised views. These are very cool things. They do them well too. It’s a shame that they’re locked in a world dislocated from general consumer programs.
There are also a few things missing, like databases don’t really do events, streams, messaging, whatever you want to call it. Some newer ones do, but none cover what you might call ‘general purpose’ streams. This means the query-driven paradigm often leaks into the application space. Applications end up circling around centralised mutable state. Whilst there are valid use cases for this, the rigid and synchronous world produced can be counterproductive for many types of programs.
So it’s interesting to look at the pros and cons of externalising caches, indexes, materialised views and asynchronous streams of state. I got to see some of these ideas play out in a data platform built for a large financial institution. It used messaging as the system of record. It also employed synchronous and asynchronous views. These could be generated, and regenerated, from this event stream.
The approach had some nice side effects which we didn’t originally anticipate. Making the system of record an event stream was actually born somewhat from necessity. The front of the system used a data grid as a consolidation point. Data grids need external persistence. We wanted the back end to support analytics. Analytic systems work best when writes are batched, so we needed something to buffer the two. Something that would scale out disk writes linearly with the sharded data grid. Topic based messaging seemed like a good fit. Clients needed notifications anyway.
This led to some interesting properties. The front end provided a near term, consistent view. Clients could collaborate around it. It could be scaled out horizontally by adding shards. At the back, everything was asynchronous and immutable. This meant it was easy to scale with replicas. Creating another replica is relatively simple when your system of record is an event stream.
The event stream tied the two together. It was the bridge between the operational and analytic sections. A stream for clients to fork directly or as a firehose to create another view.
So this is a pattern that bridges operational and analytic worlds. The operational layer provides state management for recent data. The stream buffers these changes as a log. The log forms a replayable, immutable, chronology. Views are created via functions that operate on the stream. The flow of versions is ubiquitous and unidirectional. This creates a nice synergy between stream and query. Data in motion and data at rest just become different points of reference.
After a couple of years a few things became apparent. The first was that scaling the consistent layer at the front was harder than replicating at the back. The problem was that most users ran fairly complex queries, rather than doing key-based access. General queries & processing doesn’t scale out linearly in a shared nothing model, particularly when you get to hundreds of nodes. Only key based access has that grace. You can scale out, but you get diminishing returns as you grow.
The views at the back were, by contrast, easier to scale, at least for simple analytics, ad hoc queries and report-style stuff which big organisations have lots of. This was quite nice.
At it’s essence this is just CQRS. But it’s partial CQRS. Writes at the front are separated from reads at the back. But if a writer needs to read the current state, say to support conditional changes or non-commutative actions, then a mutable view is available*. Conversely the back end leverages the benefits of an immutable, append only world.
So this offloads reads from the contended front section. But it also means the back section can ‘specialise’. This is what you might refer to as a set of materialised views or indexes. Different data arrangements, with different populations, in different places, using different indexing strategies or even different technologies altogether.
Now there are other good ways to achieve this. Simple database replication (relationally or nosql) is a good route. Relational folk would do this with the data mart pattern. Some newer products, particularly nosql ones (Mongo etc), provide both replication and sharding as first class citizens, meaning a single technology can provide a good proportion of this function out of the box. But it’s harder to get truly broad utility from a single product. These days we often want to combine a range of search, analytic, relational and routable (selector based) messaging technologies to leverage their respective sweet spots.
So integrating a set of technologies into a single data platform helps play to such functional sweet spots whilst making the problems of polyglotic persistence more manageable.
An important element of this is the ability to generate, regenerate and widen views, on demand, from the original stream. This is analogous to the way a database creates materialised views, changing them as you alter the view definition. Hadoop pipelines often do this too, in one form or another. But if you don’t address this holistically problems will likely ensue. You’ll end up altering views independently, in an ad hoc manner, rather than appending to, and replaying, the stream. This leads down a path of divergence. Pain will follow.
So the trick, at least for me, is how this is all tied together. A synchronous writeable view at the front. A range of different read-only views at the back, running asynchronous to one another. An event stream tying it all together with a single journal of state. Side effect free functions that (re)generate different views from the stream. A spout for programs to listen and interact. All wrapped up in a single data platform. A single joined up unit.
Martin suggests, in his original talk, using the Samza stack to manage views like these with Kafka providing the log. This seems a good place to start today. Kafka’s bare-boned approach certainly removes many of the scalability barriers seen in JMS/AMQP implementations, albeit at the cost of some utility.
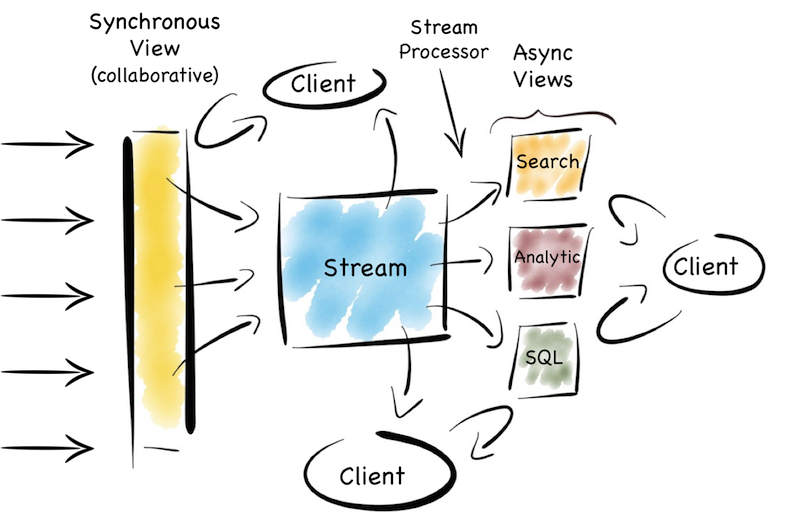
There are, of course, a plethora of little devils lurking in the detail. There are also a number of points that I skimmed over here. I’m not sure that I’d use a data grid again. In fact I’d argue that the single collaboration point isn’t always necessary*. Time synchronisation across asynchronous views can cause problems. Replaying functions on a historical stream of state is also pretty tricky, particularly as time passes and inbound data formats change. This deserves a post of its own. Finally scaling traditional messaging systems, even using topics, becomes painful, particularly when message selectors are used.
So a solution of this type needs a fairly beefy use case to warrant the effort needed to sew it all together. You wouldn’t use it for a small web app, but it would work well for a large team, division or small company. Ours was a fairly hefty central data programme. But it’s easier today than it was five years ago, and it’ll get easier still. Of that I’m sure.
So it seems unlikely we’ll go back to writing entire applications inside a ‘database’. The world doesn’t really work so well that way. Thankfully, it seems even less likely we’ll go back to writing applications in SQL. But having infrastructure that leverages the separation of mutable and immutable state, synchronicity and asynchronicity. That synergises stream and query. That is a good thing. That gets us to a better place. That, I think, is a pretty nice place to be.
—/—
* Whether you need a fast or consistent, collaborative view, to manage stateful changes, is something worth carefully considering. Many use cases simply collect, process and produce a result. That’s to say they avoid updates, and can live, instead, in a world of immutable values. This means they can neglect the consistent, temporal context needed to update data. This is a fairly deep topic so all I’ll say here is, if you can make do with an append only, immutable data, avoid having a synchronous, consistent view.
Best of VLDB 2014
Sunday, March 8th, 2015
REWIND: Recovery Write-Ahead System for In-Memory Non-Volatile Data-Structures
Interesting paper on write ahead logs in persistent in memory media. Recent non-volatile memory (NVM) technologies, such as PCM, STT-MRAM and ReRAM, can act as both main memory and storage. This has led to research into NVM programming models, where persistent data structures remain in memory and are accessed directly through CPU loads and stores. REWIND outperforms state-of-the-art approaches for data structure recoverability as well as general purpose and NVM-aware DBMS-based recovery schemes by up to two orders of magnitude.
Asterix is an academically established hierarchical store. It’s now an Apache Incubator project. It utilises sets of LSM structures, tied transactionally together. Additional index structures can also be formed, for example R-Trees.
Staring into the Abyss: An Evaluation of Concurrency Control with One Thousand Cores
As the number of cores increases, the complexity of coordinating competing accesses to data will likely diminish the gains from increased core counts.We conclude that rather than pursuing incremental solutions, many-core chips may require a completely redesigned DBMS architecture that is built from ground up and is tightly coupled with the hardware.
E-Store: Fine-Grained Elastic Partitioning for Distributed Transaction Processing Systems
OLTP DBMS need to be elastic; that is, they must be able to expand and contract resources in response to load fluctuations and dynamically balance load as hot tuples vary over time. This paper presents E-Store, an elastic partitioning framework for distributed OLTP DBMSs. It automatically scales resources in response to demand spikes, periodic events, and gradual changes in an application’s workload. E-Store addresses localized bottlenecks through a two-tier data placement strategy: cold data is distributed in large chunks, while smaller ranges of hot tuples are assigned explicitly to individual nodes. This is in contrast to traditional single-tier hash and range partitioning strategies.
Large-Scale Distributed Graph Computing Systems
An Experimental EvaluationGood coverage of different systems for distributed graph computation, including reflection on why some work better than others. Interesting.
Faster Set Intersection with SIMD instructions by Reducing Branch Mispredictions
This paper describes our new algorithm to efficiently find set intersections with sorted arrays on modern processors with SIMD instructions and high branch misprediction penalties. The key insight for our improvement is that we can reduce the number of costly hard-to-predict conditional branches by advancing a pointer by more than one element at a time. Although this algorithm increases the total number of comparisons, we can execute these comparisons more efficiently using the SIMD instructions and gain the benefits of the reduced branch misprediction overhead. Also see Improving Main Memory Hash Joins on Intel Xeon Phi Processors: An Experimental Approach
A new hash tables for joins, and a hash join based on them, that consumes far less memory and is usually faster than recently published in-memory joins. The join mechanism is not restricted to outer tables that fit wholly in memory. The key to this hash join is a new concise hash table (CHT), a linear probing hash table that has 100% fill factor, and uses a sparse bitmap with embedded population counts to almost entirely avoid collisions. This bitmap also serves as a Bloom filter for use in multi-table joins. Our experiments show that we can reduce the memory usage by one to three orders of magnitude, while also being competitive in performance.
General Incremental Sliding-Window Aggregation
This paper presents Reactive Aggregator (RA), a new framework for incremental sliding-window aggregation. RA is general in that it does not require aggregation functions to be invertible or commutative, and it does not require windows to be FIFO. We implemented RA as a drop-in replacement for the Aggregate operator of a commercial streaming engine.
Persistent B+-Trees in Non-Volatile Main Memory
A look at the application of B+- trees optimisation to provide efficient retrieval algorithms for Phase Change Memory structures.
Understanding the Causes of Consistency Anomalies in Apache Cassandra
A recent paper on benchmarking eventual consistency showed that when a constant workload is applied against Cassandra, the staleness of values returned by read operations exhibits interesting but unexplained variations when plotted against time. In this paper we reproduce this phenomenon and investigate in greater depth the low-level mechanisms that give rise to stale reads. We show that the staleness spikes exhibited by Cassandra are strongly correlated with garbage collection, particularly the “stop-the-world” phase which pauses all application threads in a Java virtual machine. We show experimentally that the staleness spikes can be virtually eliminated by delaying read operations artificially at servers immediately after a garbage collection pause. In our experiments this yields more than a 98% reduction in the number of consistency anomalies that exceed 5ms, and has negligible impact on throughput and latency.
MRCSI: Compressing and Searching String Collections with Multiple References
Efficiently storing and searching collections of similar strings, such as large populations of genomes or long change histories of documents from Wikis, is a timely and challenging problem. We then propose three heuristics for computing Multi-Reference Compressed Search Indexes, achieving increasing compression ratios. Compared to state-of-the-art competitors, our methods target an interesting and novel sweet-spot between high compression ratio versus search efficiency.
Log Structured Merge Trees
Saturday, February 14th, 2015
It’s nearly a decade since Google released its ‘Big Table’ paper. One of the many cool aspects of that paper was the file organisation it uses. The approach is more generally known as the Log Structured Merge Tree, after this 1996 paper, although the algorithm described there differs quite significantly from most real-world implementations.
LSM is now used in a number of products as the main file organisation strategy. HBase, Cassandra, LevelDB, SQLite, even MongoDB 3.0 comes with an optional LSM engine, after it’s acquisition of Wired Tiger.
What makes LSM trees interesting is their departure from binary tree style file organisations that have dominated the space for decades. LSM seems almost counter intuitive when you first look at it, only making sense when you closely consider how files work in modern, memory heavy systems.
Some Background
In a nutshell LSM trees are designed to provide better write throughput than traditional B+ tree or ISAM approaches. They do this by removing the need to perform dispersed, update-in-place operations.
So why is this a good idea? At its core it’s the old problem of disks being slow for random operations, but fast when accessed sequentially. A gulf exists between these two types of access, regardless of whether the disk is magnetic or solid state or even, although to a lesser extent, main memory.
The figures in this ACM report here/here make the point well. They show that, somewhat counter intuitively, sequential disk access is faster than randomly accessing main memory. More relevantly they also show sequential access to disk, be it magnetic or SSD, to be at least three orders of magnitude faster than random IO. This means random operations are to be avoided. Sequential access is well worth designing for.
So with this in mind lets consider a little thought experiment: if we are interested in write throughput, what is the best method to use? A good starting point is to simply append data to a file. This approach, often termed logging, journaling or a heap file, is fully sequential so provides very fast write performance equivalent to theoretical disk speeds (typically 200-300MB/s per drive).
Benefiting from both simplicity and performance log/journal based approaches have rightfully become popular in many big data tools. Yet they have an obvious downside. Reading arbitrary data from a log will be far more time consuming than writing to it, involving a reverse chronological scan, until the required key is found.
This means logs are only really applicable to simple workloads, where data is either accessed in its entirety, as in the write-ahead log of most databases, or by a known offset, as in simple messaging products like Kafka.
So we need more than just a journal to efficiently perform more complex read workloads like key based access or a range search. Broadly speaking there are four approaches that can help us here: binary search, hash, B+ or external.
- Search Sorted File: save data to a file, sorted by key. If data has defined widths use Binary search. If not use a page index + scan.
- Hash: split the data into buckets using a hash function, which can later be used to direct reads.
- B+: use navigable file organisation such as a B+ tree, ISAM etc.
- External file: leave the data as a log/heap and create a separate hash or tree index into it.
All these approaches improve read performance significantly ( n->O(log(n)) in most). Alas these structures add order and that order impedes write performance, so our high speed journal file is lost along the way. You can’t have your cake and eat it I guess.
An insight that is worth making is that all four of the above options impose some form of overarching structure on the data.
Data is deliberately and specifically placed around the file system so the index can quickly find it again later. It’s this structure that makes navigation quick. Alas the structure must of course be honoured as data is written. This is where we start to degrade write performance by adding in random disk access.
There are a couple of specific issues. Two IOs are needed for each write, one to read the page and one to write it back. This wasn’t the case with our log/journal file which could do it in one.
Worse though, we now need to update the structure of the hash or B+ index. This means updating specific parts of the file system. This is known as update-in-place and requires slow, random IO. This point is important: in-place approaches like this scatter-gun the file system performing update-in-place*. This is limiting.
One common solution is to use approach (4) A index into a journal – but keep the index in memory. So, for example, a Hash Table can be used to map keys to the position (offset) of the latest value in a journal file (log). This approach actually works pretty well as it compartmentalises random IO to something relatively small: the key-to-offset mapping, held in memory. Looking up a value is then only a single IO.
On the other hand there are scalability limits, particularly if you have lots of small values. If your values were just say simple numbers then the index would be larger than the data file itself. Despite this the pattern is a sensible compromise which is used in many products from Riak through to Oracle Coherence.
So this brings us on to Log Structured Merge Trees. LSMs take a different approach to the four above. They can be fully disk-centric, requiring little in memory storage for efficiency, but also hang onto much of the write performance we would tie to a simple journal file. The one downside is slightly poorer read performance when compared to say a B+Tree.
In essence they do everything they can to make disk access sequential. No scatter-guns here!
*A number of tree structures exist which do not require update-in-place. Most popular is the append-only Btree, also know as the copy-on-write tree. These work by overwriting the tree structure, sequentially, at the end of the file each time a write occurs. Relevant parts of the old tree structure, including the top level node, are orphaned. Through this method update-in-place is avoided as the tree sequentially redefines itself over time. This method does however come at the cost: rewriting the structure on every write is verbose. It creates a significant amount of write amplification which is a downside unto itself.
The Base LSM Algorithm
Conceptually the base LSM tree is fairly simple. Instead of having one big index structure (which will either scatter-gun the file system or add significant write amplification) batches of writes are saved, sequentially, to a set of smaller index files. So each file contains a batch of changes covering a short period of time. Each file is sorted before it is written so searching it later will be fast. Files are immutable; they are never updated. New updates go into new files. Reads inspect all files. Periodically files are merged together to keep the number of files down.
Lets look at this in a little more detail. When updates arrive they are added to an in-memory buffer, which is usually held as a tree (Red-Black etc) to preserve key-ordering. This ‘memtable’ is replicated on disk as a write-ahead-log in most implementations, simply for recovery purposes. When the memtable fills the sorted data is flushed to a new file on disk. This process repeats as more and more writes come in. Importantly the system is only doing sequential IO as files are not edited. New entries or edits simply create successive files (see fig above).
So as more data comes into the system, more and more of these immutable, ordered files are created. Each one representing a small, chronological subset of changes, held sorted.
As old files are not updated duplicate entries are created to supersede previous records (or removal markers). This creates some redundancy initially.
Periodically the system performs a compaction. Compaction selects multiple files and merges them together, removing any duplicated updates or deletions (more on how this works later). This is important both to remove the aforementioned redundancy but, more importantly, to keep a handle on the read performance which degrades as the number of files increases. Thankfully, because the files are sorted, the process of merging the files is quite efficient.
When a read operation is requested the system first checks the in memory buffer (memtable). If the key is not found the various files will be inspected one by one, in reverse chronological order, until the key is found. Each file is held sorted so it is navigable. However reads will become slower and slower as the number of files increases, as each one needs to be inspected. This is a problem.
So reads in LSM trees are slower than their in-place brethren. Fortunately there are a couple of tricks which can make the pattern performant. The most common approach is to hold a page-index in memory. This provides a lookup which gets you ‘close’ to your target key. You scan from there as the data is sorted. LevelDB, RocksDB and BigTable do this with a block-index held at the end of each file. This often works better than straight binary search as it allows the use of variable length fields and is better suited to compressed data.
Even with per-file indexes read operations will still slow as the number of files increases. This is kept in check by periodically merging files together. Such compactions keep the number of files, and hence read performance, within acceptable bounds.
Even with compaction reads will still need to visit many files. Most implementations void this through the use of a Bloom filter. Bloom filters are a memory efficient way of working out whether a file contains a key.
So from a ‘write’ perspective; all writes are batched up and written only in sequential chunks. There is an additional, periodic IO penalty from compaction rounds. Reads however have the potential to touch a large number of files when looking up a single row (i.e. scatter-gun on read). This is simply the way the algorithm works. We’re trading random IO on write for random IO on read. This trade off is sensible if we can use software tricks like bloom filters or hardware tricks like large file caches to optimise read performance.
Basic Compaction
To keep LSM reads relatively fast it’s important to manage-down the number of files, so lets look more deeply at compaction. The process is a bit like generational garbage collection:
When a certain number of files have been created, say five files, each with 10 rows, they are merged into a single file, with 50 rows (or maybe slightly less) .
This process continues with more 10 row files being created. These are merged into 50 row files every time the fifth file fills up.
Eventually there are five 50 row files. At this point the five 50 row files are merged into one 250 row file. The process continues creating larger and larger files. See fig.
The aforementioned issue with this general approach is the large number of files that are created: all must be searched, individually, to read a result (at least in the worst case).
Levelled Compaction
Newer implementations, such as those in LevelDB, RocksDB and Cassandra, address this problem by implementing a level-based, rather than size-based, approach to compaction. This reduces the number of files that must be consulted for the worst case read, as well as reducing the relative impact of a single compaction.
This level-based approach has two key differences compared to the base approach above:
1. Each level can contain a number of files and is guaranteed, as a whole, to not have overlapping keys within it. That is to say the keys are partitioned across the available files. Thus to find a key in a certain level only one file needs to be consulted.
The first level is a special case where the above property does not hold. Keys can span multiple files.
2. Files are merged into upper levels one file at a time. As a level fills, a single file is plucked from it and merged into the level above creating space for more data to be added. This is slightly different to the base-approach where several similarly sized files are merged into a single, larger one.
These changes mean the level-based approach spreads the impact of compaction over time as well as requiring less total space. It also has better read performance. However the total IO is higher for most workloads meaning some of the simpler write-oriented workloads will not see benefit.
Summarising
So LSM trees sit in the middle-ground between a journal/log file and a traditional single-fixed-index such as a B+ tree or Hash index. They provide a mechanism for managing a set of smaller, individual index files.
By managing a group of indexes, rather than a single one, the LSM method trades the expensive random IO associated with update-in-place in B+ or Hash indexes for fast, sequential IO.
The price being paid is that reads have to address a large number of index files rather than just the one. Also there is additional IO cost for compaction.
If that’s still a little murky there are some other good descriptions here and here.
Thoughts on the LSM approach
So are LSM approaches really better than traditional single-tree based ones?
We’ve seen that LSM’s have better write performance albeit a cost. LSM has some other benefits though. The SSTables (the sorted files) a LSM tree creates are immutable. This makes the locking semantics over them much simpler. Generally the only resource that is contended is the memtable. This is in contrast to singular trees which require elaborate locking mechanisms to manage changes at different levels.
So ultimately the question is likely to be about how write-oriented expected workloads are. If you care about write performance the savings LSM gives are likely to be a big deal. The big internet companies seem pretty settled on this subject. Yahoo, for example, reports a steady progression from read-heavy to read-write workloads, driven largely by the increased ingestion of event logs and mobile data. Many traditional database products still seem to favour more read-optimised file structures though.
As with Log Structured file systems [see footnote] the key argument stems from the increasing availability of memory. With more memory available reads are naturally optimised through large file caches provided by the operating system. Write performance (which memory doesn’t improve with more) thus becomes the dominant concern. So put another way, hardware advances are doing more for read performance than they are for writes. Thus it makes sense to select a write-optimised file structure.
Certainly LSM implementations such as LevelDB and Cassandra regularly provide better write performance than single-tree based approaches (here and here respectively).
Beyond Levelled LSM
There has been a fair bit of further work building on the LSM approach. Yahoo developed a system called Pnuts which combines LSM with B trees and demonstrates better performance. I haven’t seen openly available implementations of this algorithm though. IBM and Google have done more recent work in a similar vein, albeit via a different path. There are also related approaches which have similar properties but retain an overarching structure. These include Fractal Trees and Stratified Trees.
This is of course just one alternative. Databases utilise a huge range of subtly different options. An increasing number of databases offer pluggable engines for different workloads. Parquet is a popular alternative for HDFS and pushes in pretty much the opposite direction (aggregation performance via a columnar format). MySQL has a storage abstraction which is pluggable with a number of different engines such as Toku‘s fractal tree based index. This is also available for MongoDB. Mongo 3.0 includes the Wired Tiger engine which provides both B+ & LSM approaches along with the legacy engine. Many relational databases have configurable index structures that utilise different file organisations.
It’s also worth considering the hardware being used. Expensive solid state disks, like FusionIO, have better random write performance. This suits update-in-place approaches. Cheaper SSDs and mechanical drives are better suited to LSM. LSM’s avoid the small random access patters that thrash SSDs into oblivion**.
LSM is not without it critics though. It’s biggest problem, like GC, is the collection phases and the effect they have on precious IO. There is an interesting discussion of some of these on this hacker news thread.
So if you’re looking at data products, be it BDB vs. LevelDb, Cassandra vs. MongoDb you may tie some proportion of their relative performance back to the file structures they use. Measurements appear to back this philosophy. Certainly it’s worth being aware of the performance tradeoffs being selected by the systems you use.
**In SSDs each write incurs a clear-rewrite cycle for a whole 512K block. Thus small writes can induce a disproportionate amount of churn on the drive. With fixed limits on block rewrites this can significantly affect their life.
Further Reading
- There is a nice introductory post here.
- The LSM description in this paper is great and it also discusses interesting extensions.
- These three posts provide a holistic coverage of the algorithm: here, here and here.
- The original Log Structured Merge Tree paper here. It is a little hard to follow in my opinion.
- The Big Table paper here is excellent.
- LSM vs Fractal Trees on High Scalability.
- Recent work on Diff-Index which builds on the LSM concept.
- Jay on SSDs and the benefits of LSM
- Interesting discussion on hackernews regarding index structures.
Footnote on log structured file systems
Other than the name, and a focus on write throughput, there isn’t that much relation between LSM and log structured file systems as far as I can see.
Regular filesystems used today tend to be ‘Journaling’, for example ext3, ext4, HFS etc are tree-based approaches. A fixed height tree of inodes represent the directory structure and a journal is used to protect against failure conditions. In these implementations the journal is logical, meaning it only internal metadata will be journaled. This is for performance reasons.
Log structured file systems are widely used on flash media as they have less write amplification. They are getting more press too as file caching starts to dominate read workloads in more general situations and write performance is becoming more critical.
In log structured file systems data is written only once, directly to a journal which is represented as a chronologically advancing buffer. The buffer is garbage collected periodically to remove redundant writes. Like LSM’s the log structured file system will write faster, but read slower than its dual-writing, tree based counterpart. Again this is acceptable where there is lots of RAM available to feed the file cache or the media doesn’t deal well with update in place, as is the case with flash.
List of Database/BigData Benchmarks
Friday, February 13th, 2015
I did some research at the end of last year looking at the relative performance of different types of databases: key value, Hadoop, NoSQL, relational.
I’ve started a collaborative list of the various benchmarks I came across. There are many! Checkout below and contribute if you know of any more (link).
Building a Career in Technology
Friday, January 2nd, 2015
I was asked to talk to some young technologists about about their career path in technology. These are my notes which wander somewhat between career and general advice.
- Don’t assume progress means a career into management – unless you really love management. If you do, great, do that. You’ll get paid well, but it will come with downsides too. Focus on what you enjoy.
- Don’t confuse management with autonomy or power, it alone will give you neither. If you work in a company, you will always have a boss. The value you provide to the company gives you autonomy. Power comes mostly from the respect others have for you. Leadership and management are not synonymous. Be valuable by doing things that you love.
- Practice communicating your ideas. Blog, convince friends, colleagues, use github, whatever. If you want to change things you need to communicate your ideas, finding ways to reach your different audiences. If you spot something that seems wrong, try to change it by both communicating and by doing.
- Sometimes things don’t come off the way you expect. Normally there is something good in there anyway. This is ok.
- The T-shaped people idea from the Valve handbook is a good way to think about your personal development. Have a specialty, but don’t be monomaniacal. What’s your heavy weaponry?
- Whatever speciality you find yourself in, start by really knowing the fundamentals. Dig deep sooner rather than later as knowledge compounds.
- Always have a side project bubbling along. Something that’s not directly part of your job. Go to a hack night, learn a new language, teach yourself to paint, whatever, it doesn’t need to be vocational, just something that broadens your horizons and keeps you learning.
- If you find yourself thinking any particular technology is the best thing since sliced bread, and it’s somewhere near a top of the Gartner hype-curve, you are probably not seeing the full picture yet. Be critical of your own opinions and look for bias in yourself.
- Pick projects that where you have some implicit physical or tactical advantage over the competition. Don’t assume that you will do a better job just because you are smarter.
- In my experience the most telling characteristic of a good company is that its employee’s assume their colleagues are smart people, implicitly trusting their opinions and capabilities until explicitly proven otherwise. If the modus operandi of a company (or worse, a team) is ‘everyone else is an idiot’ look elsewhere.
- If you’re motivated to do something, try to capitalise on that motivation there and then and enjoy the productivity that comes with it. Motivation is your most precious commodity.
- If you find yourself supervising people, always think of their success as your success and work to maximise that. Smart people don’t need to be controlled, they need to be enabled.
- Learn to control your reaction to negative situations. The term ‘well-adjusted’ means exactly that. It’s not an innate skill. Start with email. Never press send if you feel angry or slighted. In tricky situations stick purely to facts and remove all subjective or emotional content. Let the tricky situation diffuse organically. Doing this face to face takes more practice as you need to notice the onset of stress and then cage your reaction, but the rules are the same (stick to facts, avoid emotional language, let it diffuse).
- If you offend someone always apologies. Always. Even if you are right, it is unlikely your intention was to offend.
- Recognise the difference between being politically right and emotionally right. As humans we’re great at creating plausible rationalisations and justifications for our actions, both to ourselves and others. Making such rationalisations is often a ‘sign’ of us covering an emotional mistake. Learn to notice these signs in yourself. Look past them to your moral compass.
A Guide to building a Central, Consolidated Data Store for a Company
Tuesday, December 2nd, 2014
Quite a few companies are looking at some form of centralised operational store, data warehouse, or analytics platform. The company I work for set out to build a centralised scale-out operational store using NoSQL technologies five or six years ago and it’s been an interesting journey. The technology landscape has changed a lot in that time, particularly around analytics, although that was not our main focus (but it will be an area of growth). Having an operational store that is used by many teams is, in many ways, harder than an analytics platform as there is a greater need for real-time consistency. The below is essentially a brain dump on the subject.
On Inter-System (Enterprise) Architecture
- Assuming you use some governed enterprise messaging schema: if it ends up just being an intermediary for replicating from one database to another, then you’ll be in for trouble in the long run (see here). Make something the system of record. Replicate that as a stream of changes. Ideally, make it a database replication stream via goldengate or similar so it’s exactly what is in the source database.
- Following this – clone data from a database transaction log, rather than extracting it and manually transforming to a wire format. The problem here is the word ‘manually’.
- Prefer direct access at source. Use data virtualisation if you can get it to work.
- Federated approaches, which leave data sets in place, will get you there faster if you can get all the different parts of the company to conform. That itself is a big ask though, but a good technical strategy can help. Expect to spend a lot on operations and automation lining disparate datasets up with one another.
- When standardising the persisted representation don’t create a single schema upfront if you can help it. You’ll end up in EDW paralysis. Evolve to it over time.
- Start with disparate data models and converge them incrementally over time using schema-on-need (and yes you can do this relationally, it’s just a bit harder).
On building a Multi-tenanted Read-Write store
- Your goal, in essence, is to appear like a single store from the outside but with performance and flexibility that simulates (or is) a dedicated instance for each customer. It works best to think federated from the start and build centralisation in, not the other way around.
- Utilise off the shelf products as much as possible. NoSQL products, in particular, are often better suited to this use case, but know their limitations too (see technology choice later)
- Do everything you can to frame your use case to not be everything a traditional database has to do, at least holistically, but you will probably end up having to do the majority of it anyway, at least from the point of view of a single customer.
- Question whether you really need a synchronous consolidation point for writes. It’s much easier to scale asynchronous replicas.
- Use both sharding and read-replicas to manage query load. Sharding scales key-value read and write throughput linearly, replication scales complex (non-key-value) query load linearly (at the cost of write performance if it’s synchronous). You need primitives for both sharding and replication to scale non-trivial workloads.
- Help yourself by grouping actors as either Read-Only or Read-Write for different data sets. Read-Only users can generally operate on an asynchronous dataset. This removes them from the global-write-path and hence avoids them contributing to the bottleneck that forms around it. Again, question whether you really need a single consolidation point for writes.
- This is important enough to restate: leverage both sharding and replication (both sync and async). Make async the default. Use sharding + synchronous replicas to scale complex query load on read-write datasets. Use async for everything else. Place replicas on different hardware to provide resource isolation. Use this to create a ‘store-of-stores’ model that mixes replicas (for workload isolation) with sharding (for scale out in a replica).
- Include a single event stream (log); one that exactly represents the entire stream of state. This should serve both as your asynchronous replication stream, but also as a basis for notification so you can listen as easily as you can query.
- If you provide notifications on asynchronous replicas use a proxy, located on each replica, to republish events so that the read and notification ‘views’ line up temporally. This allows clients to listen to the replicas they are reading from without race conditions between events and data being present in a replica. A prerequisite for this is consistent timestamping (covered below).
- Leverage schema-on-need. This is a powerful concept for a centralised store as it provides an incremental approach for conformity. It gets you there far faster than schema-upfront approaches. I cannot overstate how useful this is and is the backing for concepts like Data Lake etc.
- However, if you take the schemaless/on-need route be warned: historic data will need to be ‘migrated’ as the schema of the data changes, else programs will have to support different schemas or simply won’t work with old data. You can do this with different sets of ‘late-bindings’ but the eventually migration is always needed so make sure you’re tooled up for this.
- So… provision a mechanism for schema migration, else new programs won’t work with older data (note that schema migration doesn’t imply an upfront schema. It does imply a schema for each entity type though).
- Accept that all non-freeform data has a schema itself, whether you declare it up-front, on-need or not-at-all.
- Leverage the difference between query-schema and data-schema (query- being a subset of the data-schema) to minimise coupling to the store itself (all stores which utilise indexes will need a query schema as a minimum).
- Even if you are schemaless, grow some mandatory schema over time, as data stabilises. Schemas make it much easier for you to manage change when you have more customers.
- Whether you have a schema or not, data will change in a non-backwardly compatible way over time. Support this with two schemas (or data sets) visible concurrently to allow customers to upgrade using a rolling window rather than individual, lock-step releases.
- If you have to transform data on the way in, keep hold of the original in the store and give it the same key/versioning/timestamping so you can refer back to it. Make this original form a first class citizen.
- Apply the single writer principal wherever possible so only one source masters a certain dataset. Often this won’t be possible but do it wherever you can. It will allow you to localise/isolate their consistency concerns and leverage asynchronicity where consumption is off the write path.
- Don’t mix async inputs (e.g. messages that overwrite) with synchronous updates (edits) on the same entity. At best people will get very confused. If you have to mix them, hold them separately and version each. Expose this difference between these updates/overwrites on your API so they can at least be specified declaratively to the user.
- Leverage the fact that, in a collaborative store, atomaticity is a requirement but consistency can be varied. That is to say that different observers (readers not writers) can lock into different temporal points and see an atomic stream of changes. This alleviates the need for a single, global synchronisation on read. This only works if systems don’t message one another directly, else you’ll get race conditions but releases you from global transactional locks and that’s often worth the tradeoff, particularly if you’re crossing data-centre boundaries.
- Make data immutable. Timestamp and version everything (validFrom-validTo etc). Build this into the very core of the system. Flag the latest record so you don’t always have to read all versions to get the latest. Keep all changes if you can afford the storage. It will allow you to look back in time. But know that temporal indexes are the least-efficient / most-complex-to-manage index type (generally require temporal partitioning).
- Applying time consistently in a distributed environment requires synchronisation on a central clock (don’t rely on NTP). As a central clock is not always desirable, consider using epochs (consistent periods) which are pushed to each node so to define global read-consistent periods without explicit synchronisation (but at a courser granularity of ‘tick’). See here.
- Don’t fall into the relational trap of splitting entities just because they are polystructured and don’t fit in a single row. Hold an entity separately only where real world entities they represented vary independently.
- In tension with that, don’t denormalise data from different sources on the write path (i.e. using aggregates), if those aggregates contain many->1 relationships that do change independently. It will make writing atomically more difficult as writes must update multiple denormalised entities. Prefer joins to bring together the data at runtime or use aggregates in reporting replicas.
- Provide, as a minimum, multi-key transactions in the context of a master replica. This will require synchronisation of writes, but it is possible to avoid synchronisation of reads.
- Access to the data should be declarative (don’t fall into the trap of appending methods to an API to add new functionality). Make requests declarative. Use SQL (or a subset of) if you can.
- Know that building a platform used by different teams is much harder than building a system. Much of the extra difficulty comes from peripheral concerns like testing, automation, reliability, SLAs that compound as the number of customers grow.
- Following from this think about the customer development lifecycle early, not just your production system. Make environments self-service. Remember data movement is network limited and datasets will be large.
- Testing will hurt you more and more as you grow. Provide system-replay functions to make testing faster.
- Your value in a company is based mostly on a perception of your overall value. If you’re doing a platform-based data consolidation project you will inevitably restrict what they can do somewhat. Focus on marketing and support to keep your users happy.
On Technology Choice
- Use off the shelf products as much as possible.
- The relational model is good for data you author but not so good for data sourced from elsewhere (data tends to arrive polystructured so is best stored polystructured).
- Be wary of pure in-memory products and impressive RAM-centric benchmarks. Note their performance as data expands onto disk. You always end up with more data than you expect and write amplification is always more than vendors state.
- Accept that, despite the attraction of a polystructured approach, the relational model is a necessity in most companies, at least for reporting workloads, so the wealth of software designed to work with it (and people skilled in it) can be leveraged.
- NoSQL technologies are stronger in a number of areas, most notably:
- scalability for simple workloads,
- polystructured data,
- replication primitives,
- continuous availability,
- complex programmable analytics.
- Relational technologies are stronger at:
- keeping data usable for evolving programs over long periods of time,
- transactional changes,
- pre-defined schemas,
- breadth of query function,
- analytical query performance.
- In effect this ends up being: use the right tool for the right job, refer to 5/6 with as few technologies as you can survive with.
Useful talk on Linux Performance Tools
Sunday, August 24th, 2014
http://www.brendangregg.com/
An initial look at Actian’s ‘SQL in Hadoop’
Monday, August 4th, 2014
I had an exploratory chat with Actian today about their new product ‘SQL in Hadoop’.
In short it’s a distributed database which runs on HDFS. The company are bundling their DataFlow technology alongside this. DataFlow is a GUI-driven integration and analytics tool (think suite of connectors, some distributed functions and a GUI to sew it all together).
Neglecting the DataFlow piece for now, SQL in Hadoop has some obvious strengths. The engine is essentially Vectorwise (recently renamed ‘Vector’). A clever, single node columnar database which evolved from MonetDB and touts the use of vectorisation as a key part of its secret sauce. Along with the usual columnar benefits comes the use of positional delta trees which improve on the poor update performance seen in most columnar databases, some clever cooperative scan technology which was presented at VLDB a couple of years back, but they don’t seem to tout this one directly. Most notably Vector has always had quite impressive benchmarks both in absolute and price-performance terms. I’ve always thought of it as the relational analytics tool I’d look to if I were picking up the tab.
The Vector engine (termed x100) is the core of Actian’s SQL in Hadoop platform. It’s been reengineered to use HDFS as its storage layer, which one has to assume will allow it to operate better price performance when compared to storage-array based MPPs. It has also had a distribution layer placed above it to manage distributed queries. This appears to leverage parts of the DataFlow cluster as well as Yarn and some other elements of the standard Hadoop stack. It inherits some aspects of traditional MPPs such as the use of replication to improve data locality over declared, foreign key, join conditions. The file format in HDFS is wholly proprietary though so it can’t be introspected directly by other tools.
So whilst it can be deployed inside an existing Hadoop ecosystem, the only benefit gained from the Hadoop stack, from a user’s perspective, is the use of HDFS and YARN. There is no mechanism for integrating MR or other tools directly with the x100 engines. As the file format is opaque the database must be addressed as an SQL appliance even from elsewhere within the Hadoop ecosystem.
Another oddity is that, by branching Vector into the distributed world the product directly competes with its sister product Matrix (a.k.a. ParAccel); another fairly accomplished MPP which Actian acquired a couple of years ago. If nothing else this leads to a website that is pretty confusing.
So is the product worth consideration?
It’s most natural competition must be Impala. Impala however abstracts itself away from the storage format, meaning it can theoretically operate on any data in HDFS, although from what I can tell all practical applications appear to transform source files to something better tuned for navigation (most notably parquet). Impala thus has the benefit that it will play nicely with other areas of the Hadoop stack, Hive, Hbase etc. You won’t get any of this with the Actian SQL in Hadoop product although nothing is to stop you running these tools alongside Actian on Hadoop, inside the same HDFS cluster.
Actian’s answer to this may be the use of its DataFlow product to widen the appeal to non-sql workloads as well as data ingestion and transformation tasks. The DataFlow UI does provide a high level abstraction for sewing together flows. I’ve always been suspicious of how effective this is for non-trivial workflows which generally involve the authoring of custom adapters and functions, but it obviously has some traction.
A more natural comparison might come from other MPPs such as Greenplum, which offers a HDFS integrated version and ties in to the Pivotal Hadoop distribution. Comparisons with other MPPs, Paraccel, Netazza, Vertica etc are also reasonable if you are not restricted to HDFS.
So we may really be looking at a tradeoff between the breadth of the OS Hadoop stack vs. SQL compliance & raw performance. The benefits of playing entirely in a single ecosystem, like the Cloudera offering, is a better integration between the tools, an open source stack which has a rich developer community, less issues of vendor lock-in and a much broader ecosystem of players (Drill, Storm, Spark, Skoop and many more).
Actian on the other hand can leverage its database heritage; efficient support the full SQL spec, ACID transactions and a performance baseline that comes from a relatively mature data warehousing offering where aggregation performance was the dominant driver.
As a full ecosystem it is probably fair to say it lacks maturity at this time, certainly when compared to Hadoop’s. In the absence of native connectivity with other Hadoop products it is really a database appliance on HDFS rather than a 1st class entity in the Hadoop world. But there are certainly companies pushing for better performance than they can currently get on existing HDFS infrastructure with the core Hadoop ecosystem. For this Actian’s product could provide a useful tool.
In reality the proof will be in the benchmarks. Actian claim order of magnitude performance improvements over Impala. Hadapt, an SQL on Hadoop startup which was backed by and ex-Vertica/academic partnership was hammered by market pressure from Impala and was eventually bought by Teradata. The point being that the performance needs to justify breaking out of the core ecosystem.
There may be a different market though in companies with relationally-centric users looking to capitalise on the cheaper storage offered by HDFS. This would also aid, or possibly form a strategy away from siloed, SAN based products and towards the broadly touted (if somewhat overhyped) merits of Big Data and commodity hardware on the Cloud. Hype aside that could have considerable financial benefit.
Edit: Peter Boncz, who is one of the academics behind the original vector product, published an informative review with benchmarks here. There is also an academic overview of (a precursor to) the system here. Worth a read.
A little more on ODC
Tuesday, June 3rd, 2014
We’re hiring! so I thought I’d share this image which shows a little more about what ODC is today. There is still a whole lot to do but we’re getting there.
Metrics (the good and the bad):
- Loc: 300k
- Tests: 27k
- Build time 33mins
- Engineering team (3uk, 10 India)
- Coverage 70% (85% in core database)
There is a little more about the system here. If you’re interested in joining us give me a shout.
ODC Core Engineer: Interested in pure engineering work using large data sets where performance is the primary concern? ODC provides a unique solution for scaling data using a blend NoSQL and Relational concepts in a single store. This is a pure engineering role, working on the core product. Experience with performance optimisation, distributed systems and strong Computer Science is useful.
ODC Automation Engineer: Do you enjoy automation, pulling tricky problems together behind a veneer of simplicity? Making everything in ODC self-service is one of the our core goals. Using tools like Groovy, Silver Fabric and Cloudify you will concentrate on automating all operational aspects of ODC’s core products, integrating with RBS Cloud to create a data-platform-as-a-service. Strong engineering background, Groovy and automation experience (puppet, chef, cloudify etc) preferred but not essential.
Using Airplay as an alternative to Sonos for multi-room home audio
Wednesday, May 28th, 2014
I chose not to go down the Sonos route for the audio in my flat. This was largely because I wanted sound integrated sound in five rooms, wanted to reuse some of the kit I already had, didn’t want to fork out Sonos prices (Sonos quote was pushing £2k and I ended up spending about £100 on the core setup) and because I kinda liked the idea of building something rather than buying it in.
This actually took quite a bit of fiddling around to get right so this post covers where I’ve got to so far.
I live in a flat (~150sqm) and have installed 6 linked speakers over five rooms. Two in the lounge and one in the kitchen, bathroom, study, wardrobe.
The below describes the setup and how to get it all to play nicely:
– Like Sonos, you need a media server to coordinate everything and it really needs to be physically wired to your router. I use an old 2007 macbook pro, configured with NoSleep and power-saving disabled, so it keeps running when the lid’s closed.
– The server is connected to the router via a homeplug. This is pretty important as it effectively halves your dependence on wireless which is inevitably flakey, particularly if you live in a built up area or use you microwave much. Wiring the server to the router had a big affect on the stability of the system.
– The server runs Airfoil (I tried a number of others including Portholefor a while but Airfoil appears to be the most stable and functional).
For the 6 speakers I use:
– My main hifi (study) is plugged directly into the Macbook server through the audio jack.
– A really old mac mini (which can only manage Snow Loepard) in the lounge is connected to my other stereo using the free Airfoil speakers app on OSX. Airfoil itself won’t run on Snow Leopard so this wasn’t an option for the server, but the Airfoil speaker app works fine.
– Canton Musicbox Air – an expensive piece of kit I bought on a bit of a whim. It definitely sounds good, but it cost twice the price of everything else put together so I’m not sure I’d buy it again.
– Two bargain basement, heavily reduced airplay units. The killer feature of doing it yourself is that airplay speakers are a few years old now and there are quite a few decent ones out there for around £50. I use a Philips Fidelio AD7000W which is nice and thin (it sits in a kitchen cupboard), has impressive sound for its size and only cost £40 on amazon marketplace (a second). Also a Pure Contour 200i which cost £50. This goes pretty loud although I find the sound a little muffled. I certainly prefer the crispness of the Fidelio despite less bass. The Contour is also the only unit I’ve found with an ethernet port (useful as you can add a homeplug, but I found this wasn’t necessary once the media server was attached to the router, unless the microwave is on). I should add that both of these are heavily reduced at the moment because they are getting old and the Contour has the old-style, 30pin iphone dock on it – it’s also kinda ugly so I have it tucked away.
– Iphone 3 connected to some Bose computer speakers I had already. The Iphone runs the free Airfoil speaker app. One annoying thing about the Iphone app is that if Airfoil is restarted on the server the iphone doesn’t automatically reconnect, you have to go tell it to. I don’t restart the server much so it’s not really a problem but I’ll replace it with a raspberry pi at some point.
– Finally you need to control it all. This is the bit where Sonos has the edge. There is no single, one-stop-shop app for all your music needs (that I’ve found anyway). I control everything from my Iphone 5, listening mostly to ITunes and Spotify so the closest to a one-stop-shop is Remoteless for Spotify. This allows you almost complete control of Spotify but it’s not as good as the native Spotify app. It does however let you control Airfoil too so you can stop and start speakers, control volume and move between different audio sources. It also has apps for a range of media sources (Pandora, Deezer etc). When sourcing from ITunes I switch to the good old ITunes Remote App and use this to play my music library as well as Intenet radio. Also of note are Airfoil Remote (nice interface for controlling Airfoil itself but it’s ability to control apps is very limited) and Spot Remote which is largely similar to Remoteless but without the Airfoil integration.
So is it worth it??
OK so this is the bargain basement option. It’s not as slick as the real thing. However, all round, it works pretty well. The Sonos alternative system, which would have involved 2 Sonos bridges (for the two existing stereos), Play 3’s for the each of the periphery rooms and a play 5 in the lounge, would have pushed two grand. Discounting my splurge on the Conton, the system described here is about £100. In honesty though I quite enjoy the fiddling 😉
A note on interference and cut outs: These happen from time to time. Maybe once a week or so. I did a fair bit of analysis on my home network with Wifi Explorer and Wire Shark. Having a good signal around the place is obviously important. I use a repeater, connected via a homeplug. The most significant change, regarding dropouts, was to connect the server to the router via a homeplug. Since then dropouts are very rare.
If you don’t fancy the faff, you want something seamless and money isn’t an issue I’d stick with Sonos, it just works. Also it hasn’t passed the girlfriend test. She still connects her iphone directly to each of the airplay units. However if you don’t mind this, fancy something a little more DIY and have a few bits and bobs lying around the Airplay route works for a fraction of the price.
Sizing Coherence Indexes
Monday, April 28th, 2014
This post suggests three ways to measure index sizes in Coherence: (a)Using the Coherence MBean (not recommended) (b) Use JMX to GC the cluster (ideally programatically) as you add/remove indexes – this is intrusive (c) Use the SizeOf invocable (recommended) – this requires teh use of a -javaagent option on your command line.
(a) The Coherence MBean
Calculating the data size is pretty easy, you just add a unit calculator and sum over the cluster (there is code to do that here: test, util). Indexes however are more tricky.
Coherence provides an IndexInfo MBean that tries to calculate the size. This is such an important factor in maintaining you cluster it’s worth investigating.
Alas the IndexInfo Footprint is not very accurate. There is a test,IsCoherenceFootprintMBeanAccurate.java,which demonstrates there are huge differences in some cases (5 orders of magnitude). In summary:
– The Footprint is broadly accurate for fairly large fields (~1k) where the index is unique.
– As the cardinality of the index drops the Footprint Mbean starts to underestimate the footprint.
– As the size of the field being indexed gets smaller the MBean starts to underestimate the index.
Probably most importantly for the most likely case, for example the indexed fields is fairly small say 8B, and the cardinality is around half the count, the MBean estimate is out by three orders of magnitude.
Here are the results for the cardinality of half the count and field sizes 8B, 16B, 32B
Ran: 32,768 x 32B fields [1,024KB indexable data], Cardinality of 512 [512 entries in index, each containing 64 values], Coherence MBean measured: 49,152B. JVM increase: 3,162,544B. Difference: 6334% Ran: 65,536 x 16B fields [1,024KB indexable data], Cardinality of 512 [512 entries in index, each containing 128 values], Coherence MBean measured: 40,960B. JVM increase: 5,095,888B. Difference: 12341% Ran: 131,072 x 8B fields [1,024KB indexable data], Cardinality of 512 [512 entries in index, each containing 256 values], Coherence MBean measured: 40,960B. JVM increase: 10,196,616B. Difference: 24794%
In short, it’s too inaccurate to be useful.
(b) Using JMX to GC before and after adding indexes
So the we’re left with a more intrusive process to work out our index sizes:
- Load your cluster up with indexes.
- GC a node and take it’s memory footprint via JMX/JConsole/VisualVm
- Drop all indexes
- GC the node again and work out how much the heap dropped by.
I have a script which does this programatically via JMX. It cycles through all the indexes we have doing:
ForEach(Index) { GC->MeasureMemory->DropIndex->GC->MeasureMemory->AddIndexBack }This method works pretty well although it takes a fair while to run if you have a large number of indexes, and is intrusive so you couldn’t run in production. It also relies on our indexes all being statically declared in a single place. This is generally a good idea for any project. I don’t know of a way to extract the ValueExtractor programatically from Coherence so just use the static instance in our code.
(c) Use Java’s Inbuilt Instrumentation
This is the best way (in my opinion). It’s simple and accurate. The only issue is that you need to start your coherence processes with a javaagent as it’s using the Instrumentation API to size indexes.
The instrumentation agent itself is very simple, and uses the library found here. All we need to wrap that is an invocable which executes it on each node in the cluster.
The invocable just loops over each cache service, and each cache within that service, calculating the size of the IndexMap using the instrumentation SizeOf.jar
To implement this yourself:
1) Grab these two classes: SizeOfIndexSizer.java, IndexCountingInvocable.java and add them to your classpath. The first sets the invocable off, handling the results. The second is the invocable that runs on each node and calculates the size of the index map.
2) Take a copy of SizeOf.jar from here and add -javaagent:<pathtojar>/SizeOf.jar to your command line.
3) Call the relevant method on SizeOfIndexSizer.
When is POF a Good Idea?
Saturday, April 12th, 2014
POF is pretty cool. Like Protocol Buffers, which they are broadly similar to, POF provides an space-efficient, byte-packed wire / storage format which is navigable in its binary form. This makes it a better than Java serialisation for most applications (although if you’re not using Coherence then PB are a better bet).
Being a bit-packed format it’s important to understand the performance implications of extracting different parts of the POF stream. This being different to the performance characteristics of other storage formats, in particular fixed width formats such as those used in most databases which provide very fast traversal.
To get an understanding of the POF format see the primer here. In summary:
1. Smaller than standard Java Serialisation: The serialised format is much smaller than java serialisation as only integers are encoded in the stream rather than the fully class/type information.
2. Smaller than fixed-width formats: The bit-packed format provides a small memory footprint when compared to fixed length fields and doesn’t suffer from requiring overflow mechanisms for large values. This makes it versatile.
3. Navigable: The stream can be navigated to read single values without deserialising the whole stream (object graph).
Things to Watch Out For:
1. Access to fields further down the stream is O(n) and this can become dominant for large objects:
Because the stream is ‘packed’, rather than using fixed length fields, traversing the stream is O(n), particularly the further down the stream you go. That’s to say extracting the last element will be slower than extracting the first. Fixed width fields have access times O(1) as they can navigate to a field number directly.
We can measure this using something along the lines of:
Binary pof = ExternalizableHelper.toBinary(object, context); SimplePofPath path = new SimplePofPath(fieldPos);//vary the position in the stream PofExtractor pofExtractor = new PofExtractor(ComplexPofObject.class, path); while (count --> 0) { PofValue value = PofValueParser.parse(pof, context); pofExtractor.getNavigator().navigate(value).getValue(); }
If you want to run this yourself it’s available here: howMuchSlowerIsPullingDataFromTheEndOfTheStreamRatherThanTheStart(). This code produces the following output:
> Extraction time for SimplePofPath(indices=1) is 200 ns > Extraction time for SimplePofPath(indices=2) is 212 ns > Extraction time for SimplePofPath(indices=4) is 258 ns > Extraction time for SimplePofPath(indices=8) is 353 ns > Extraction time for SimplePofPath(indices=16) is 564 ns > Extraction time for SimplePofPath(indices=32) is 946 ns > Extraction time for SimplePofPath(indices=64) is 1,708 ns > Extraction time for SimplePofPath(indices=128) is 3,459 ns > Extraction time for SimplePofPath(indices=256) is 6,829 ns > Extraction time for SimplePofPath(indices=512) is 13,595 ns > Extraction time for SimplePofPath(indices=1024) is 27,155 ns
It’s pretty clear (and not really surprising) that the navigation goes O(n). The bigger problem is that this can have an affect on your queries as your datasize grows.
Having 100 fields in a pof object is not unusual, but if you do, the core part of your query is going to run 20 slower when retrieving the last field than it is when you retrieve the first.
For a 100 field object, querying on the 100th field will be 20 times slower than querying the first
This is just a factor of the variable length encoding. The code has no context of the position of a particular field in the stream when it starts traversing it. It has no option but to traverse each value, find it’s length and skip to the next one. Thus the 10th field is found by skipping the first 9 fields. This is in comparison to fixed length formats where extracting the nth field is always O(1).
2) It can be more efficient to deserialise the whole object, and makes your code simpler too
If you’re just using a simple filter (without an index) POF makes a lot of sense, use it, but if you’re doing more complex work that uses multiple fields from the stream then it can be faster to deserialise the whole object. It also makes your code a lot simpler as dealing with POF directly gets pretty ugly as the complexity grows.
We can reason about whether it’s worth deserialising the whole object by comparing serialisation times with the time taken to extract multiple fields.
The test whenDoesPofExtractionStopsBeingMoreEfficient() measures the break even point beyond which we may as well deserialise the whole object. Very broadly speaking it’s 4 extractions, but lets look at the details.
Running the test yields the following output:
On average full deserialisation of a 50 field object took 3225.0ns On average POF extraction of first 5 fields of 50 took 1545.0ns On average POF extraction of last 5 fields of 50 took 4802.0ns On average POF extraction of random 5 fields of 50 took 2934.0ns
Running this test and varying the number of fields in the object leads to the following conclusions.
– for objects of 5 fields the break even point is deserialising 2 pof fields
– for objects of 20 fields the break even point is deserialising 4 pof fields
– for objects of 50 fields the break even point is deserialising 5 pof fields
– for objects of 100 fields the break even point is deserialising 7 pof fields
– for objects of 200 fields the break even point is deserialising 9 pof fields
Or to put it another way, if you have 20 fields in your object and you extracted them one at a time it would be five times slower than deserialising.
In theory the use of the PofValue object should optimise this. The PofValueParser, which is used to create PofValue objects, effectively creates an index over the pof stream meaning that, in theory, reading multiple fields from the pof value should be O(1) each. However in these test I have been unable to see this gain.
Conclusions/Recommendations
Pof is about more than performance. It negates the need to put your classes (which can change) on the server. This in itself is a pretty darn good reason to use it. However it’s worth considering performance. It’s definitely faster than Java serialisation. That’s a given. But you do need to be wary about using pof extractors to get individual fields, particularly if you have large objects.
The degradation is O(n), where n is the number of fields in the object, as the stream must be traversed one field at a time. This is a classic space/time tradeoff. The alternative, faster O(1), fixed-width approach would require more storage which can be costly for in memory technologies.
Fortunately there is a workaround of sorts. If you have large objects, and are using POF extraction for your queries (i.e. you are not using indexes which ensure a deserialised field will be on the heap), then prefer composites of objects to large (long) flat ones. This will reduce the number of skipPofValue() calls that the extractor will have to do.
If you have large objects and are extracting many fields to do their work (more than 5-10 extractions per object) then it may be best to deserialise the whole thing. In cases like this pof-extraction will be counter productive, at least from a performance perspective. Probably more importantly, if you’re doing 5-10 extractions per object, you are doing something fairly complex (but this certainly happens in Coherence projects) so deserialising the object and writing your logic against PoJos is going to make your code look a whole lot better too. If in doubt, measure it!
Ref: JK posted on this too when we first became aware of the problem.
POF Primer
Saturday, April 12th, 2014
This is a brief primer on POF (Portable Object Format) used in Coherence to serialise data. POF is much like Google’s Protocol Buffers so if you’re familiar with those you probably don’t need to read this.
POF a variable length, bit-packed serialisation format used to represent object graphs as byte arrays in as few bytes as possible, without the use of compression. Pof’s key property is that it is navigable. That is to say you can pull a value (object or primitive) out of the stream without having to deserilalise the whole thing. A feature that is very useful if you want to query a field in an object which is not indexed.
The Format
Conceptually simple, each class writes out its fields to a binary stream using a single bit-packed (variable length encoded) integer as an index followed by a value. Various other pieces of metadata are also encoded into the stream using bit-packed ints. It’s simplest to show in pictorially:
Variable Length Encoding using Bit-Packed Values
Variable length encoding uses as few bytes as possible to represent a field. It’s worth focusing on this for a second. Consider the job of representing an Integer in as few bytes as possible. Integers are typically four bytes but you don’t really need four bytes to represent the number 4. You can do that in a few bits.
PackedInts in Coherence take advantage of this to represents an integer in one to five bytes. The first bit of every byte indicates whether subsequent bytes are needed to represent this number. The second bit of the first byte represents the sign of the number. This means there are six ‘useful’ bits in the first byte and 7 ‘useful’ bits in all subsequent ones, where ‘useful’ means ‘can be used to represent our number’.
Taking an example let’s look at representing the number 25 (11001) as a bit-packed stream:
25 //Decimal 11001 //Binary [0 0 0011001] //POF (leading bits denote: whether more bytes are needed, the sign)
Line 1 shows our decimal, line 2 its binary form. Line 3 shows how it is represented as POF. Note that we have added four zeros to the front of the number denoting that no following bytes are required to represent the number and that the number is positive.
If the number to be encoded is greater than 63 then a second byte is needed. The first bit again signifies whether further bits will be needed to encode the number. There is no sign-bit in the second byte as it’s implied from the first. Also, just to confuse us a little, the encoding of the numeric value is different to the single-byte encoding used above: the binary number is reversed so the least significant byte is first (the whole number appears reversed across the two bytes). So the number 128 (10000000) would be encoded:
128 //Decimal 10000000 //Binary 00000001 //Binary (reversed) 000000 00010 //Aligned [1 0 000000] [0 00010] //POF
The pattern continues with numbers greater than or equal to 2^13 which need a third byte to represent them. For example 123456 (11110001001000000) would be represented
123456 //Decimal 11110001001000000 //Binary 00000010010001111 //Reversed 000000 1001000 0001111 //Aligned [1 0 000000] [1 0001001] [0 0001111] //POF
Note again that the binary number is reversed and then laid with the least significant bit first (unlike the single btye encoding above).
In this way the average storage is as small as it can be without actually using compression.
Exploring a POF Stream (see Gist)
We can explore a little further by looking at the Coherence API. Lets start with a simple POF object:
public class PofObject implements PortableObject { private Object data; PofObject(Object data) { this.data = data; } public void readExternal(PofReader pofReader) throws IOException { data = pofReader.readObject(1); } public void writeExternal(PofWriter pofWriter) throws IOException { pofWriter.writeObject(1, data); } }
We can explore each element in the stream using the readPackedInt() method to read POF integers and we’ll need a readSafeUTF() for the String value:
SimplePofContext context = new SimplePofContext(); context.registerUserType(1042, PofObject.class, new PortableObjectSerializer(1042)); PofObject object = new PofObject("TheData"); //get the binary & stream Binary binary = ExternalizableHelper.toBinary(object, context); ReadBuffer.BufferInput stream = binary.getBufferInput(); System.out.printf("Header btye: %s\n" + "ClassType is: %s\n" + "ClassVersion is: %s\n" + "FieldPofId is: %s\n" + "Field data type is: %s\n" + "Field length is: %s\n", stream.readPackedInt(), stream.readPackedInt(), stream.readPackedInt(), stream.readPackedInt(), stream.readPackedInt(), stream.readPackedInt() ); System.out.printf("Field Value is: %s\n", binary.toBinary(6, "TheData".length() + 1).getBufferInput().readSafeUTF() );
Running this code yields:
> Header btye: 21 > ClassType is: 1042 > ClassVersion is: 0 > FieldPofId is: 1 > Field data type is: -15 > Field length is: 7 > Field Value is: TheData
Notice line 25, which reads the UTF String, requires the length as well as the value (it reads bytes 6-15 where 6 is the length and 7-15 are the value).
Finally POF Objects are nested into the stream. So if field 3 is a user’s object, rather than a primitive value, an equivalent POF-stream for the user’s object is nested in the ‘value’ section of the stream, forming a tree that represents the whole object graph.
The code for this is available on Gist and there is more about POF internals in the coherence-bootstrap project on github: PofInternals.java.
Transactions in KV stores
Tuesday, February 25th, 2014
Something close to my own heart – interesting paper on lightweight milti-key transactions for KV stores.
http://hyperdex.org/papers/warp.pdf
Scaling Data Slides from EEP
Tuesday, February 4th, 2014
A little bit of Clojure
Friday, November 15th, 2013
Slides for today’s talk at RBS Techstock:
Slides from JAX London
Friday, November 1st, 2013
Similar name to the Big Data 2013 but a very different deck:
The Return of Big Iron? (Big Data 2013)
Wednesday, March 27th, 2013
Slides from Advanced Databases Lecture 27/11/12
Wednesday, November 28th, 2012
The slides from yesterday’s guest lecture on NoSQL, NewSQL and Big Data can be found here.
Big Data & the Enterprise
Thursday, November 22nd, 2012
Slides from today’s European Trading Architecture Summit 2012 are here.
Problems with Feature Branches
Saturday, November 10th, 2012
Over the last few years we’ve had a fair few discussions around the various different ways to branch and how they fit into a world of Continuous Integration (and more recently Continuous Delivery). It’s so fundamental that it’s worth a post of its own!
Dave Farley (the man that literally wrote the book on it) penned a the best advice I’ve seen on the topic a while back. Worth a read, or even a reread (and gets better towards the end).
http://www.davefarley.net/?p=160 (in case dave’s somewhat flakey site is down again the article is republished here)
Where does Big Data meet Big Database
Friday, August 17th, 2012
InfoQ published the video for my Where does Big Data meet Big Database talk at QCon this year.
Thoughts appreciated.
A Brief Summary of the NoSQL World
Saturday, August 11th, 2012
James Phillips (co-founder of Couchbase) did a nice talk on NoSQL Databases at QCon:
Memcached – the simplest and original. Pure key value store. Memory focussed
Redis – Extends the simple map-like semantic with extensions that allow the manipulation of certain specific data structures, stored as values. So there are operations for manipulating values as lists, queues etc. Redis is primarily memory focussed.
Membase – extends the membached approach to include persistence, the ability to add nodes, backup’s on other nodes.
Couchbase – a cross between Membase and CouchDB. Membase on the front, Couch DB on the back. The addition of CouchDB means you can can store and reflect on more complex documents (in JSON). To query Couchbase you need to write javascript mapping functions that effectively materialise the schema (think index) so that you can create a query model. Couchbase is CA not AP (i.e. not eventually consistent)
MongoDB – Uses BSON (binary version of JSON which is open source but only really used by Mongo). Mongo unlike the Couchbase in that the query language is dynamic: Mongo doesn’t require the declaration of indexes. This makes it better at adhoc analysis but slightly weaker from a production perspective.
Cassandra – Column oriented, key value. The value are split into columns which are pre-indexed before the information can be retrieved. Eventually consistent (unlike Couchbase). This makes it better for highly distributed use cases or ones where the data is spread over an unreliable networks.
Neo4J – Graph oriented database. Much more niche. Not distributed.
There are obviously a few more that could have been covered (Voldemort, Dynamo etc but a good summary from James none the less)
Full slides/video can be found here.
Looking at Intel Xeon Phi (Kinghts Corner)
Thursday, August 9th, 2012
Characteristics:
- Intel’s new MIC ‘Knights Corner’ coprocessor (in the Intel Xeon Phi line) is targeted at the high concurrency market, previously dominated by GPGPUs, but without the need for code to be rewritten into Cuda etc (note Knights Ferry is the older prototype version).
- The chip has 64 cores and 8GBs of RAM with a 512b vector engine. Clock speed is ~ 1.1Ghz and have a 512k L1 cache. The linux kernel runs on two 2.2GHZ processors.
- It comes on a card that drops into a PCI slot so machines can install multiple units.
- It uses a MESI protocol for cache coherence.
- There is a slimmed down linux OS that can run on the processor.
- Code must be compiled to two binaries, one for the main processor and one for Knights Corner.
- Compilers are currently available only for C+ and Fortran. Only Intel compilers at present.
- It’s on the cusp of being released (Q4 this year) for NDA partners (though we – GBM – have access to one off-site at Maidenhead). Due to be announced at the Supercomputing conference in November(?).
- KC is 4-6 GFLOPS/W – which works out at 0.85-1.8 TFLOPS for double precision.
- It is expected to be GA Q1 ’13.
- It’s a large ‘device’ the wafer is a 70mm square form-factor!
- Access to a separate board over PCI is a temporary step. Expected that future versions will be a tightly-coupled co-processor. This will also be on the back of the move to the 14nm process.
- A single host can (depending on OEM design) support several PCI cards.
- Similarly power-draw and heat-dispersal an OEM decision.
- Reduced instruction set e.g. no VM support instructions or context-switch optimisations.
- Performance now being expressed as GFlops per Watt. This is a result of US Government (efficiency) requirements.
- A single machine is can go faster than a room-filling supercomputer of ’97 – ASIC_Red!
- The main constraint to doing even more has been the limited volume production pipeline.
- Pricing not announced, but expected to be ‘consistent with’ GPGPUs.
- Key goal is to make programming it ‘easy’ or rather: a lot easier than the platform dedicated approaches or abstraction mechanisms such as OpenCL.
- Once booted (probably by a push of an OS image from the main host’s store to the device) it can appear as a distinct host over the network.
Commentary:
- The key point is that Knights Corner provides most of the advantages of a GPGPU but without the painful and costly exercise of migrating software from one language to another (that is to say it is based on the familiar x86 programming model).
- Offloading work to the card is instructed through the offload pragma or offloading keywords via shared virtual memory.
- Computation occurs in a heterogeneous environment that spans both the main CPU and the MIC card which is how execution can be performed with minimal code changes.
- There is a reduced instruction set for Knights Corner but the majority of the x86 instructions are there.
- There is support for OpenCl although Intel are not recommending that route to customers due to performance constraints.
- Real world testing has shown a provisional 4x improviement in throughput using an early version of the card running some real programs. However results from a sample test shows perfect scaling. Some restructuring of the code was necessary. Not huge but not insignificant.
- There is currently only C++ and Fortran interfaces (so not much use if you’re running Java or C#)
- You need to remember that you are on PCI Express so you don’t have the memory bandwidth you might want.
References:
Other things worth thinking about:
Thanks to Mark Atwell for his help with this post.
Progressive Architectures at RBS
Friday, July 6th, 2012
Michael Stal wrote a nice article about the our Progressive Architectures talk from this year’s QCon. The video is up too.
Read the InfoQ article here.
Watch the QCon video here.
A big thanks to Fuzz, Mark and Ciaran for making this happen.
Harvey Raja’s ‘Pof Art’ Slides
Friday, June 15th, 2012
I really enjoyed Harvey’s ‘POF Art’ talk at the Coherence SIG. Slides are here if you’re into that kind of thing POF-Art.
Simply Being Helpful?
Wednesday, May 30th, 2012
What if, more than anything else, we valued helping each other out? What if this was the ultimate praise, not the best technologists, not an ability to hit deadlines, not production stability. What if the ultimate accolade was to consistently help others get things done? Is that crazy? It’s not always natural; we innately divide into groups, building psychological boundaries. Conflicts can erupt from trivial things. And what about the business? How would we ever deliver anything if we spent all our time helping each other out? Well maybe we’d deliver quite a lot.
If helping each other out were our default position it would simply be more efficient? We’d have less politics, less conflict, fewer empires and we’d spend less money managing them.
We probably can’t change who we are. We’ll always behave a bit like we do now. Conflict will always arise and it will always result in problems. We all have tempers, we play games, we have biases and egos. We frustrate others and react to slights and injustices.
But what if was simply our goto position. Our core value. The thing we fall back on when we’re not sure where to go. The thing we used to define our success. It wouldn’t be a silver bullet, but it might temper some of the torrid inefficiencies that dog large organisations.
… right back to the real world
Valve Handbook
Wednesday, May 16th, 2012
Valve handbook. Very cool:
loadedtrolley.com.au/Valve-
Welcome Jon ‘The Gridman’ Knight
Tuesday, January 24th, 2012
Jon ‘The Gridman’ Knight has finally dusted off his keyboard and entered the blogsphere with fantastic post on how we implement a reliable version of Coherence’s putAll() over here on ODC. One to add to your feed if you are interested in all things Coherence.
http://thegridman.com/coherence/coherence-alternative-putall-2/
Interesting Links Dec 2011
Saturday, December 31st, 2011
Hardware
- Intel managing to squeeze 50 cores on a single chip, breaking through the teraflop boundary as they do so: Brier Dudley’s Blog | Wow: Intel unveils 1 teraflop chip with 50-plus cores | Seattle Times Newspaper
- RISC architectures have had a renaissance thanks largely to the needs of the mobile sector, could their low power consumption make them a serious contender for enterprise space? x86 Faces Unexpected RISC Competition
- AMD announce 4 memory channels allowing massive addressable spaces up to 364GB per CMP : AMD’s Interlagos and Valencia finally emerge
- Anyone who follows my blog will know of my belief in large address spaces reshaping the landscape, certainly for enterprise applications. This articles echoes these views: Megatrend: Cheap RAM Reshaping All of Computing | Dr Dobb’s
FPGA
- IBM’s Lime is an interesting approach to simplifying the programming of secondary devices. See Lime paper and the related Liquid Metal project.
- JVM on FPGA: JOP: A Tiny Java Processor Core for FPGA
- An interesting paper on using FPGA for Monte Carlo Simulation: FPGA for monte carlos
High Performance Java
- An excellent talk about using memory efficiently in Java applications, that the costs are often higher than we think. It includes clear descriptions of the footprint of all Java objects and utilities : Building Memory Efficient Java Applications
- There has been a flurry of activity coming from Azul Systems recently. Most notably the release of Zing, their pauseless garbage collector. Gene Til’s talk about the State of the Art in GC from QCon SF 2011 is one of the best I’ve seen (QConSF 2011: State of the Art in Garbage Collection).
- Azul have also recently released JHiccup. An interesting utility that measures operating system stalls. Java Developer Tools: jHiccup Java Performance Analysis
- Charles Nutter’s comments on his favourite JVM flags including my favourite (-XX:+PrintOptoAssembly): Headius: My Favorite Hotspot JVM Flags
Distributed Data Storage
- A great paper from VLDB describing an approach for balancing replication and partitioning, something close to my own heart: Schism: a Workload-Driven Approach to Database Replication and Partitioning
- Hasso Plattner (the P is SAP) wrote this paper which provides an insigntful view of where he believes the field should be going (and of course SAP’s solution Hana): Hasso Plattner on In-Memory OLAP & OLTP
- I enjoyed watching this talk about Mongo: InfoQ: Scaling with MongoDB
Interesting:
- An entertaining article from the Economist about David Gelernter’s predictions of the future of computing: Brain scan: Seer of the mirror world | The Economist
- Could Prezi really dislodge PowerPoint? Prezi
- Double Loop Learning – a different view on organizational learning. Chris Argyris.
- Worth reading if you are not familiar with the idea already: CQRS
- An interesting twist on the traditional storyboard approach Our Story Board is Better Than Yours… I’m a big fan of replacing estimation with uniformly sized stories.
- Booked your next holiday? What about a Code Retreat with Corey Haines
Interesting Links Oct 2011
Tuesday, October 25th, 2011
High Performance Java
- Not exactly lightweight reading but one of the most detailed and influential papers on tuning your software for processing efficiency: What Developers Should Understand About Memory
- If you read the above and want to put some of it into action then VTune should be your next port of call. Diagnostic software for CPU cache hits etc: VTune™ Amplifier XE 2011 from Intel – Intel® Software Network
- When it really won’t go any faster, look at the Assembler: Deep dive into assembly code from Java | Java.net
- In anticipation of G1 (in case they ever get it finished) here’s the original paper with anticipated performance figures: G1 paper with figures
- A different approach to GC using processor specific minor collections (in Haskell): Multicore Garbage Collection with Local Heaps
Distributed Data Storage:
- The new Oracle NoSQL database – this is the best article I’ve read summarising it’s position in the market: DBMS Musings: Overview of the Oracle NoSQL Database
- The official Oracle NoSQL Whitepaper: Oracle NoSQL Database White Paper
- An interesting approach to data storage: an FPGA based data warehouse: FPGA Data Warehouse
- Google’s interesting SQL wrapped MapReduce framework: Tenzing A SQL Implementation On The MapReduce Framework
Distributed Computing:
- The Actors Model – just in case you’re not familiar with it: Actors model for distribution
- Gluster – an open source distributed file system: Gluster
- Running Cuda natively on x86 processors: Running CUDA Code Natively on x86 Processors | Dr Dobb’s Journal
Coherence related:
- Thinking about using 64bit JVMs with compressed pointers : 32-bit or 64-bit JVM? How about a Hybrid?
- Using different caches for read and write. A sensible pattern for Cohernece implementation: Alexey Ragozin’s Blog
- OCZ Z-Drive – an interesting and competitively priced alternative to FusionIO:
Just Interesting:
- The architecture of the transputer. An interesting reflection on a couple of Bristol’s finest exports (other than Portishead): the Transputer and the Occum programming language. David May, parallel processing pioneer • reghardware
- Is your brain like an Iphone? Is Your Brain Like an iPhone? Which App is Running Now? – Novato, CA Patch
- Just be still for once: No Shame in Stillness « Under the Apricot Tree
- Of the huge amount of writing about Steve Jobs I thought the Economist’s coverage was the best: Steve Jobs: The magician | The Economist
- Scott Marcar’s thought prevoking dialog on technology through a financial crisis: The Long Haul: Scott Marcar Leads RBS’ Tech Team Through the Financial Crisis- WatersTechnology.com
- Short but thought provoking article on company culture: Why You Should Question Your Culture – Ron Ashkenas – Harvard Business Review
Slides for Financial Computing course @ UCL
Sunday, October 23rd, 2011
Balancing Replication and Partitioning in a Distributed Java Database @JavaOne
Wednesday, October 5th, 2011
Here are a the slides for the talk I gave at JavaOne:
Balancing Replication and Partitioning in a Distributed Java Database
This session describes the ODC, a distributed, in-memory database built in Java that holds objects in a normalized form in a way that alleviates the traditional degradation in performance associated with joins in shared-nothing architectures. The presentation describes the two patterns that lie at the core of this model. The first is an adaptation of the Star Schema model used to hold data either replicated or partitioned data, depending on whether the data is a fact or a dimension. In the second pattern, the data store tracks arcs on the object graph to ensure that only the minimum amount of data is replicated. Through these mechanisms, almost any join can be performed across the various entities stored in the grid, without the need for key shipping or iterative wire calls.
See Also
JavaOne
Tuesday, August 9th, 2011
I’m heading to JavaOne in October to talk about some of the stuff we’ve been doing at RBS. The talk is entitled “Balancing Replication and Partitioning in a Distributed Java Database”.
Is anyone else going?
Interesting Links July 2011
Wednesday, July 20th, 2011
Because the future will inevitably be in-memory databases:
- SAP (slightly weirdly) is leading the way with Hana
- SSD makes a new kind of database possible
- The move away from clusters is not restricted to the enterprise
- More drinking of the Hana Kool-Aid
- Fusion IO
- Phase Change Memory breakthrough at IBM
Other interesting stuff:
- Interesting retrospective on computing giants of the past and future (in typical Economist style)
- A mathematician’s lament
- The next generation of Map Reduce
- Where google may be going wrong
A better way of Queuing
Monday, June 27th, 2011
The LMAX guys have open-sourced their Disruptor queue implementation. Their stats show some significant improvements (over an order of magnitude) over standard ArrayBlockingQueues in a range of concurrent tests. Both interesting and useful.
http://code.google.com/p/disruptor/
QCon Slides/Video: Beyond The Data Grid: Coherence, Normalization, Joins and Linear Scalability
Friday, June 17th, 2011
The slides/video from the my talk at QCon London have been put up on InfoQ.
http://www.infoq.com/presentations/ODC-Beyond-The-Data-Grid
The NoSQL Bible
Wednesday, April 27th, 2011
An effort well worthy of it’s own post: http://www.christof-strauch.de/nosqldbs.pdf
QCon Slides
Wednesday, March 9th, 2011
Thanks to everyone that attended the talk today at QCon London. You can find the slides here. Hard copies here too: [pdf] [ppt]
Interesting Links Feb 2011
Sunday, February 20th, 2011
Thinking local:
- Nice talk covering optimising code in a single JVM: LMAX
- Biased locking in Hotspot: biased_locking_in_hotspot
- Good overview of caching: intel-cpu-caches
- Good overview of lock free algorithms: lock-free-algorithms
Thinking Distributed:
- Nice overview of the key NoSQL players: cassandra-vs-mongodb-vs-couchdb-vs-redis
- Google’s layering of ACID over BigTable (at least ACID inside a partition):
- Original paper: CIDR11_Paper32.pdf
- Summary thereof: GoogleMegastoreTheDataEngineBehindGAE
- Commentary: comparing-google-megastore
- Commentary: a-interesting-note-on-google-megastore-cap
- Typically Economist: economist.com
QCon 2011
Tuesday, January 11th, 2011
Just a little plug for the 5th annual QCon London on March 7-11, 2011. There is a bunch of cool speakers inlcuding Craig Larman and Juergen Hoeller as well as the obligitory set of Ex-TW types. I’ll be doing a session on Going beyond the Data Grid.
You can save £100 and give £100 to charity is you book with this code: STOP100
Interesting Links Dec 2010
Monday, January 3rd, 2011
More discussions on the move to in memory storage:
- RAM is my friend
- LMAX – How to Do 100K TPS at Less than 1ms Latency
- The problems with ACID, and how to fix them without going NoSQL
- Basho Riak: An Open Source Scalable Data Store
- Facebook’s belief in HBase
- Numbers Everyone Should Know
- Google Dremel Paper
- Facebook’s New Year Performance Stats
Talk Proposal: Managing Normalised Data in a Distributed Store
Sunday, November 14th, 2010
I’ve been working on a medium sized data store (around half a TB) that provides high bandwidth and low latency access to data.
Caching and Warehousing techniques push you towards denormalisation but this becomes increasingly problematic when you move to a highly distributed environment (certainly if the data is long lived). We’ve worked on a model that is semi normalised whilst retaining the performance benefits associated with denormalisation.
The other somewhat novel attribute of the system is its use of Messaging as a system of record.
I did a talk abstract, which David Felcey from Oracle very kindly helped with, which describes the work in brief. You can find it here.
I’ll also be adding some more posts in the near future to flesh out how this all works.
Submissions being accepted for RefTest @ ICSE
Saturday, November 13th, 2010
Submissions are being accepted for RefTest at IEEE International Conference on Testing, Verification and Validation.
Submissions can be short (2 page) or full length conference papers. The deadline in Jan 4th 2011.
Full details are here.
Software Writing and the Intellectual Superiority Complex
Saturday, June 11th, 2005
Have you ever been reading something something, maybe academic, and had to pause for a moment of language-induced-brain-freeze? This is often followed by a feeling that the effort you’re putting in may not outweigh the insight that comes out! I had one of these moments when reading a book on software development failures. The piece that caught my mind was:
The postmodernist view of software development explicitly recognises the need for a plurality and diversity of shared responsibilities for all stakeholder groups involved in the development, so that all legitimate relevant views will be heard and incorporated into the problem formulation.
This is comprehensible and grammatically correct but it is just a little too obtuse to flow easily into the mind. You see this sort of thing a lot, particularly in academic circles, and wonder if such passages are more about the intellectual prowess of the author rather than the comprehension for the reader.
As a little experiment I had a go at rewriting it in the way I might if I were writing on this topic (albeit unlikely). See what you think:
Today’s methods favour stakeholders being involved in all aspects of the development process, sharing their responsibilities across the various groups involved. This is a good way to involve relevant views, from different groups, into the problem’s formulation.
I know it’s not a world away from the original but I think it is an improvement. Which do you prefer??
However the author’s passage is not ‘bad’ as such, even though it is a bit long and dense and hence a little hard to digest in one read.
I find myself asking the question; if you were writing a book aimed at helping someone learn, why write it in a way that makes it harder to understand? One answer is that of imposing intellectual prowess though the use of language, otherwise known as the assertion of intellectual superiority.
The issue of intellectual superiority seems quite prevalent in the computer sciences, possibly due to the density of technical language that we have. The breadth of domain specific acronyms and terms offers a great degree of cover to those wishing to bolster their technical position or assert intellectual superiority over others.
Yet even the use of technical language in what appears to be a legitimate context can be ill-advised. The problem is that different people interpret, and react very differently to terms that they do not understand, or maybe have a vague or partial understanding of.
For example junior members of a team will generally seek an explanation for terms they do not understand. However more senior members, management etc, are far less likely to seek similar explanations. Terms they do not understand are more likely to be ignored or partially understood. Even worse, they may be considered with suspicion!
Because of this computer scientists must be wary of their audience. Having empathy for the technical understanding of others allows domain specific terminologies to be decoded into accessible language. Staying on the same technical plain as management is important for useful two way communications to be established. Inaccessible language can damage this relationship. It also plays on a primary fear that management have of technologists, that they are somewhat pompous!
In conclusion, if you are writing a book, think about the reader rather than your ego. If you are talking to your boss, keep it simple and don’t geek them out. They are much more likely to be impressed with a concise and comprehensible comment, set at a level that they understand, than an impressive sounding, if somewhat useless, stream of techno-babble!